Warum Data Engineering der Data Science in Bedeutung und Berufschancen längst die Show stiehlt, dabei selbst ebenso einem stetigen Wandel unterliegt.
Was ein Data Engineer wirklich können muss
Der Data Scientist als sexiest Job des 21. Jahrhunderts? Mag sein, denn der Job hat seinen ganz speziellen Reiz, auch auf Grund seiner Schnittstellenfunktion zwischen Technik und Fachexpertise. Doch das Spotlight der kommenden Jahre gehört längst einem anderen Berufsbild aus der Datenwertschöpfungskette – das zeigt sich auch bei den Gehältern.
Viele Unternehmen sind gerade auf dem Weg zum Data-Driven Business, einer Unternehmensführung, die für ihre Entscheidungen auf transparente Datengrundlagen setzt und unter Einsatz von Business Intelligence, Data Science sowie der Automatisierung mit Deep Learning und RPA operative Prozesse so weit wie möglich automatisiert. Die Lösung für diese Aufgabenstellungen werden oft vor allem bei den Experten für Prozessautomatisierung und Data Science gesucht, dabei hängt der Erfolg jedoch gerade viel eher von der Beschaffung valider Datengrundlagen ab, und damit von einer ganz anderen entscheidenden Position im Workflow datengetriebener Entscheidungsprozesse, dem Data Engineer.
Data Engineer, der gefragteste Job des 21sten Jahrhunderts?
Der Job des Data Scientists hingegen ist nach wie vor unter Studenten und Absolventen der MINT-Fächer gerade so gefragt wie nie, das beweist der tägliche Ansturm der vielen Absolventen aus Studiengängen rund um die Data Science auf derartige Stellenausschreibungen. Auch mangelt es gerade gar nicht mehr so sehr an internationalen Bewerben mit Schwerpunkt auf Statistik und Machine Learning. Der solide ausgebildete und bestenfalls noch deutschsprachige Data Scientist findet sich zwar nach wie vor kaum im Angebot, doch insgesamt gute Kandidaten sind nicht mehr allzu schwer zu finden. Seit Jahren sind viele Qualifizierungsangebote für Studenten sowie Arbeitskräfte am Markt auch günstig und ganz flexibel online verfügbar, ohne dabei Abstriche bei beim Ansehen dieser Aus- und Fortbildungsmaßnahmen in Kauf nehmen zu müssen.
Was ein Data Scientist fachlich in Sachen Expertise alles abdecken muss, hatten wir ganz ausführlich über Betrachtung des Data Science Knowledge Stack besprochen.
Doch was bringt ein Data Scientist, wenn dieser gar nicht über die Daten verfügt, die für seine Aufgaben benötigt werden? Sicherlich ist die Aufgabe eines jeden Data Scientists auch die Vorbereitung und Präsentation seiner Vorhaben. Die Heranschaffung und Verwaltung großer Datenmengen in einer Enterprise-fähigen Architektur ist jedoch grundsätzlich nicht sein Schwerpunkt und oft fehlen ihm dafür auch die Berechtigungen in einer Enterprise-IT. Noch konkreter wird der Bedarf an Datenbeschaffung und -aufbereitung in der Business Intelligence, denn diese benötigt für nachhaltiges Reporting feste Strukturen wie etwa ein Data Warehouse.
Das Profil des Data Engineers: Big Data High-Tech
Auch wenn Data Engineering von Hochschulen und Fortbildungsanbietern gerade noch etwas stiefmütterlich behandelt werden, werden der Einsatz und das daraus resultierende Anforderungsprofil eines Data Engineers am Markt recht eindeutig skizziert. Einsatzszenarien für diese Dateningenieure – auch auf Deutsch eine annehmbare Benennung – sind im Kern die Erstellung von Data Warehouse und Data Lake Systeme, mittlerweile vor allem auf Cloud-Plattformen. Sie entwickeln diese für das Anzapfen von unternehmensinternen sowie -externen Datenquellen und bereiten die gewonnenen Datenmengen strukturell und inhaltlich so auf, dass diese von anderen Mitarbeitern des Unternehmens zweckmäßig genutzt werden können.
Enabler für Business Intelligence, Process Mining und Data Science
Kein Data Engineer darf den eigentlichen Verbraucher der Daten aus den Augen verlieren, für den die Daten nach allen Regeln der Kunst zusammengeführt, bereinigt und in das Zielformat gebracht werden sollen. Klassischerweise arbeiten die Engineers am Data Warehousing für Business Intelligence oder Process Mining, wofür immer mehr Event Logs benötigt werden. Ein Data Warehouse ist der unter Wasser liegende, viel größere Teil des Eisbergs der Business Intelligence (BI), der die Reports mit qualifizierten Daten versorgt. Diese Eisberg-Analogie lässt sich auch insgesamt auf das Data Engineering übertragen, der für die Endanwender am oberen Ende der Daten-Nahrungskette meistens kaum sichtbar ist, denn diese sehen nur die fertigen Analysen und nicht die dafür vorbereiteten Datentöpfe.
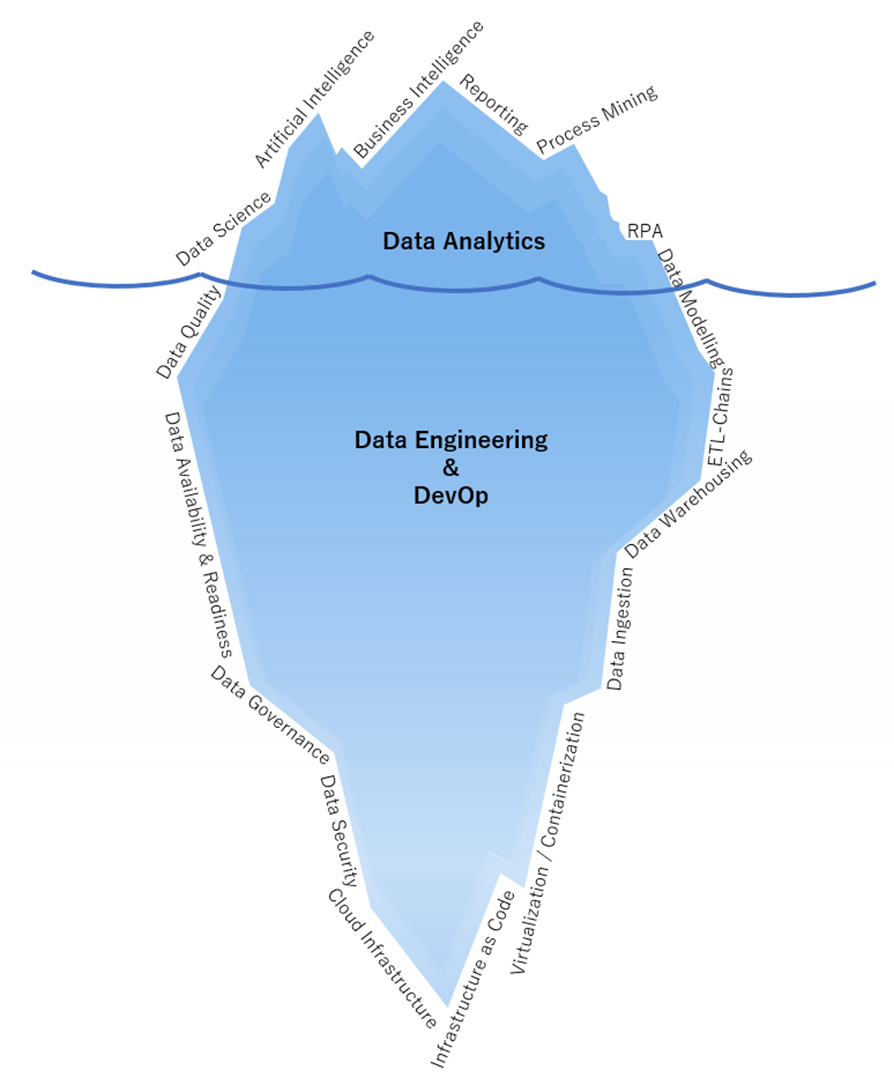
Abbildung 1 – Data Engineering ist der Mittelpunkt einer jeden Datenplattform. Egal ob für Data Science, BI, Process Mining oder sogar RPA, die Datenanlieferung bedingt gute Dateningenieure, die bis hin zur Cloud Infrastructure abtauchen können.
Datenbanken sind Quelle und Ziel der Data Engineers
Daten liegen selten direkt in einer einzigen CSV-Datei strukturiert vor, sondern entstammen einer oder mehreren Datenbanken, die ihren eigenen Regeln unterliegen. Geschäftsdaten, beispielsweise aus ERP- oder CRM-Systemen, liegen in relationalen Datenbanken vor, oftmals von Microsoft, Oracle, SAP oder als eine Open-Source-Alternative. Besonders im Trend liegen derzeitig die Cloud-nativen Datenbanken BigQuery von Google, Redshift von Amazon und Synapse von Microsoft sowie die cloud-unabhängige Datenbank snowflake. Dazu gesellen sich Datenbanken wie der PostgreSQL, Maria DB oder Microsoft SQL Server sowie CosmosDB oder einfachere Cloud-Speicher wie der Microsoft Blobstorage, Amazon S3 oder Google Cloud Storage. Welche Datenbank auch immer die passende Wahl für das Unternehmen sein mag, ohne SQL und Verständnis für normalisierte Daten läuft im Data Engineering nichts.
Andere Arten von Datenbanken, sogenannte NoSQL-Datenbanken beruhen auf Dateiformaten, einer Spalten- oder einer Graphenorientiertheit. Beispiele für verbreitete NoSQL-Datenbanken sind MongoDB, CouchDB, Cassandra oder Neo4J. Diese Datenbanken exisiteren nicht nur als Unterhaltungswert gelangweilter Nerds, sondern haben ganz konkrete Einsatzgebiete, in denen sie jeweils die beste Performance im Lesen oder Schreiben der Daten bieten.
Ein Data Engineer muss demnach mit unterschiedlichen Datenbanksystemen zurechtkommen, die teilweise auf unterschiedlichen Cloud Plattformen heimisch sind.
Data Engineers brauchen Hacker-Qualitäten
Liegen Daten in einer Datenbank vor, können Analysten mit Zugriff einfache Analysen bereits direkt auf der Datenbank ausführen. Doch wie bekommen wir die Daten in unsere speziellen Analyse-Tools? Hier muss der Engineer seinen Dienst leisten und die Daten aus der Datenbank exportieren können. Bei direkten Datenanbindungen kommen APIs, also Schnittstellen wie REST, ODBC oder JDBC ins Spiel und ein guter Data Engineer benötigt Programmierkenntnisse, bevorzugt in Python, diese APIs ansprechen zu können. Etwas Kenntnis über Socket-Verbindungen und Client-Server-Architekturen zahlt sich dabei manchmal aus. Ferner sollte jeder Data Engineer mit synchronen und asynchronen Verschlüsselungsverfahren vertraut sein, denn in der Regel wird mit vertraulichen Daten gearbeitet. Ein Mindeststandard an Sicherheit gehört zum Data Engineering und darf keinesfalls nur Datensicherheitsexperten überlassen werden, eine Affinität zu Netzwerksicherheit oder gar Penetration-Testing ist positiv zu bewerten, mindestens aber ein sauberes Berechtigungsmanagement gehört zu den Grundfähigkeiten. Viele Daten liegen nicht strukturiert in einer Datenbank vor, sondern sind sogenannte unstrukturierte oder semi-strukturierte Daten aus Dokumenten oder aus Internetquellen. Mit Methoden wie Data Web Scrapping und Data Crawling sowie der Automatisierung von Datenabrufen beweisen herausragende Data Engineers sogar echte Hacker-Qualitäten.
Dirigent der Daten: Orchestrierung von Datenflüssen
Eine der Kernaufgaben des Data Engineers ist die Entwicklung von ETL-Strecken, um Daten aus Quellen zu Extrahieren, zu in das gewünschte Zielformat zu Transformieren und schließlich in die Zieldatenbank zu Laden. Dies mag erstmal einfach klingen, wird jedoch zur echten Herausforderung, wenn viele ETL-Prozesse sich zu ganzen ETL-Ketten und -Netzwerken zusammenfügen, diese dabei trotz hochfrequentierter Datenabfrage performant laufen müssen. Die Orchestrierung der Datenflüsse kann in der Regel in mehrere Etappen unterschieden werden, von der Quelle ins Data Warehouse, zwischen den Ebenen im Data Warehouse sowie vom Data Warehouse in weiterführende Systeme, bis hin zum Zurückfließen verarbeiteter Daten in das Data Warehouse (Reverse ETL).
Hart an der Grenze zu DevOp: Automatisierung in Cloud-Architekturen
In den letzten Jahren sind Anforderungen an Data Engineers deutlich gestiegen, denn neben dem eigentlichen Verwalten von Datenbeständen und -strömen für Analysezwecke wird zunehmend erwartet, dass ein Data Engineer auch Ressourcen in der Cloud managen, mindestens jedoch die Datenbanken und ETL-Ressourcen. Darüber hinaus wird zunehmend jedoch verlangt, IT-Netzwerke zu verstehen und das ganze Zusammenspiel der Ressourcen auch als Infrastructure as Code zu automatisieren. Auch das automatisierte Deployment von Datenarchitekturen über CI/CD-Pipelines macht einen Data Engineer immer mehr zum DevOp.
Zukunfts- und Gehaltsaussichten
Im Vergleich zum Data Scientist, der besonders viel Methodenverständnis für Datenanalyse, Statistik und auch für das zu untersuchende Fachgebiet benötigt, sind Data Engineers mehr an Tools und Plattformen orientiert. Ein Data Scientist, der Deep Learning verstanden hat, kann sein Wissen zügig sowohl mit TensorFlow als auch mit PyTorch anwenden. Ein Data Engineer hingegen arbeitet intensiver mit den Tools, die sich über die Jahre viel zügiger weiterentwickeln. Ein Data Engineer für die Google Cloud wird mehr Einarbeitung benötigen, sollte er plötzlich auf AWS oder Azure arbeiten müssen.
Ein Data Engineer kann in Deutschland als Einsteiger mit guten Vorkenntnissen und erster Erfahrung mit einem Bruttojahresgehalt zwischen 45.000 und 55.000 EUR rechnen. Mehr als zwei Jahre konkrete Erfahrung im Data Engineering wird von Unternehmen gerne mit Gehältern zwischen 50.000 und 80.000 EUR revanchiert. Darüber liegen in der Regel nur die Data Architects / Datenarchitekten, die eher in großen Unternehmen zu finden sind und besonders viel Erfahrung voraussetzen. Weitere Aufstiegschancen für Data Engineers sind Berater-Karrieren oder Führungspositionen.
Wer einen Data Engineer in Festanstellung gebracht hat, darf sich jedoch nicht all zu sicher fühlen, denn Personalvermittler lauern diesen qualifizierten Fachkräften an jeder Ecke des Social Media auf. Gerade in den Metropolen wie Berlin schaffen es längst nicht alle Unternehmen, jeden Data Engineer über Jahre hinweg zu beschäftigen. Bei der großen Auswahl an Jobs und Herausforderungen fällt diesen Datenexperten nicht schwer, seine Gehaltssteigerungen durch Jobwechsel proaktiv voranzutreiben.