Es gibt Gelegenheiten, da ist eine oder mehrere serverlose Funktionen nicht ausreichend, um einen Service darzustellen. Für diese Fälle gibt es auf der Google Cloud Plattform Google Cloud Run. Cloud Run bietet zwei Möglichkeiten Container auszuführen. Services und Jobs. In diesem Beispiel wird ein Google Cloud Run Service mittels Terraform definiert, welcher auf Basis eines Scheduler Jobs regelmäßig aufgerufen wird. Cloud Build wird dazu genutzt den aktuellen Code auf den Service zu veröffentlichen.
Der nachfolgende Quellcode ist in GitHub verfügbar: https://github.com/fingineering/GCPCloudRunDemo
tl;dr
Mittels Terraform können alle notwendigen Komponenten erstellt werden, um einen Cloud Run Services aus einem Github Repository kontinuierlich zu aktualisieren. Als Beispiel wird ein stark vereinfachter Flask Webservice verwendet. Durch den Cloud Scheduler wird dieser Service regelmäßig aufgerufen.
Voraussetzungen
Bevor der Service aufgesetzt werden kann, müssen einige Voraussetzungen erfüllt sein. Es wird ein Google Cloud Project benötigt, sowie ein Github Account. Auf dem Computer, welcher zur Entwicklung verwendet werden soll, müssen Terraform, Google Cloud SDK, git, Docker und Python installiert sein. In diesem Beispiel wird Python verwendet, es ist aber mit jeder Sprache möglich, mit der ein WebServer erstellt werden kann.
- Ein Google Cloud Projekt kann bei Google Cloud Plattform erstellt werden. Es wird nur ein Google Account benötigt, Neukunden erhalten ein kostenloses Guthaben von 300€ für 90 Tage.
- Wenn noch nicht vorhanden sollte ein kostenloser Github Account auf Github erstellt werden. Das Beispiel kann auch mittels Google Cloud Source Repositories umgesetzt werden. Als Alternative zu Cloud Build kann Github Actions eingesetzt werden.
- Terraform, Google Cloud SDK, Docker und Python müssen auf dem verwendeten Computer installiert werden, hierzu empfiehlt sich ein Paketmanager wie Homebrew oder Chocolatey. Linux Nutzer verwenden am besten den in ihrer Distribution mitgelieferten.
- Soll nichts installiert werden, dann kann auch die Google Cloud Shell verwendet werden, diese findet sich im in der Cloud Console
APIs die in Google Cloud aktiviert werden müssen
Neben den Voraussetzungen zur Software müssen auf der Google Cloud Plattform einige APIs aktiviert werden:
- Cloud Run API
- Cloud Build API
- Artifact Registry API
- Cloud Scheduler API
- Cloud Logging API
- Identity and Access Management API
Die Cloud Run API wird benötigt um einen Cloud Run Service zu erstellen, die Artifact Registry wird benötigt, um die Container Abbilder zu speichern. Die Cloud Build API und die Identity and Access Management API werden benötigt, um eine CI/CD Pipeline zu implementieren. Cloud Scheduler wird in diesem Beispiel verwendet, um den Service regelmäßig aufzurufen. Da alle Services via Terraform erstellt und verwaltet werden, werden die APIs benötigt.
Infrastruktur
Das Erstellen der Infrastruktur läuft in mehreren Schritten ab, nur wenn App Code und Container bereits vorhanden sind, kann der gesamte Prozess automatisiert werden. Für die Definition der Infrastruktur wird ein Ordner “Infrastructure” erstellt und darin die Dateien main.tf
, variables.tf
und terraform.tfvars
.
|
mkdir Infrastructure cd Infrastructure touch main.tf touch terraform.tfvars |
Insgesamt werden vier Komponenten erstellt, das Artifact Registry Repository, ein Cloud Run Service, ein Cloud Build Trigger und ein Cloud Scheduler Job. Bevor die eigentliche Infrastruktur erzeugt werden kann, müssen einige Service Accounts definiert werden. Ziel ist es, das jedes Asset eine eigene Identität zugewiesen werden kann. Daher werden drei Service Accounts erstellt, für den Run Service, den Build Trigger und den Scheduler Job.
1 2 3 4 5 6 7 8 9 10 11 12 13 14 15 16 17 18 19 20 21 22
|
resource "google_service_account" "cloud_run_demo_sa" { project = var.project_name account_id = "cloudrundemosa" description = "Running Cloud Run Scheduled Job to update data in BigQuery under this account" display_name = "Cloud Run Demo Service Account" } # Service account for scheduler to call the function resource "google_service_account" "cloud_shedule_caller" { project = var.project_name account_id = "cloudscheduler" description = "A service account to run the scheduler under an invoke the cloud run service" display_name = "Cloud Scheduler Demo User" } # Service Account to run the cloud build trigger resource "google_service_account" "cloud_run_demo_builder" { project = var.project_name account_id = "cloudrundemobuilder" description = "A service account to run the cloud build trigger" display_name = "Cloud Run Demo Build Trigger" } |
Da der erste Schritt die Einrichtung der Artifact Registry für den Cloud Run Service ist, wird dieser wie folgt hinzugefügt:
|
resource "google_artifact_registry_repository" "container_demo_repo" { location = var.location project = var.project_name repository_id = var.container_name description = "Demo docker repository" format = "DOCKER" } // create the url of a container in the registry, by name of container data "google_container_registry_image" "demo_container" { name = var.container_name project = var.project_name } |
Nun kann der erste Schritt zum Aufsetzen der Infrastruktur mittels des Terraform Dreiklangs durchgeführt werden:
|
# initialize the terraform project terraform init # check if everything will go as planned terraform plan # create the infrastructure terraform apply |
Die Beispiel Flask Anwendung
Als Beispielanwendung wird hier eine sehr simple Flask Webapp verwendet. Die Webapp beinhaltet eine einzige Route, es wird Hello World
bei einem GET
Request zurück gegeben und mittels POST
kann die Nachricht personalisiert werden. Die Anwendung dient nur der Demonstration, es können fast beliebige Funktionalitäten umgesetzt werden.
Es ist auch nur zwingend notwendig flask oder Python zu verwenden, es kann jede Sprache und jedes Framework eingesetzt werden, welches einen Webserver implementieren kann und auf HTTP Anfragen reagieren kann. Flask selbst ist ein sogenanntes Micro Framework und kann flexibel eingesetzt werden, für mehr Informationen empfiehlt sich z.b. das Flask Mega Tutorial
Im Projektverzeichnis muss ein neuer Ordner App
erzeugt werden, in diesem Ordner wird die Python Datei main.py
, sowie die requirements.txt Datei erzeugt.
1 2 3 4 5 6 7 8 9 10 11 12 13 14 15 16 17 18 19 20 21 22 23 24 25 26 27
|
from flask import Flask, request app = Flask(__name__) @app.route('/', methods=['GET', 'POST']) def main(): if request.method == 'POST': # get some incomming data data = request.get_json() # This would be the place where could call your code to run. # But that wouldn't be a good idea. # Therefore we create a script package, register the scripts and run # them all in here return f"Hello {data['name']}" return "Hello World" if __name__ == '__main__': """ Creating a very basic debug app. Don't use this in production """ app.run( debug=True, port=8000, host="0.0.0.0" ) |
Dieser minimale Webservice kann local ausgeführt werden indem ein virtual environment erzeugt und die in requirements.txt spezifizierten Pakete installiert werden.
|
# create a virtual environment python -m venv venv # activate it source venv/bin/activate # install required packages pip install -r requirements.txt |
Die App kann nun local ausgeführt werden mittels:
Zum Testen kann im Browser die Adresse localhost:8080 aufgerufen werden oder mittels curl ein POST
Request an den Service gesendet werden.
|
curl -X POST -H "Content-Type: application/json" \ -d '{"name": "FooBar"}' \ http://localhost:8080 |
Der mit flask mitgelieferte Web Server sollte nur zu Entwicklungszwecken verwendet werden, in produktiven Umgebungen kann z.b. gunicorn eingesetzt werden. Gunicorn wird in diesem Beispiel später auch im Container verwendet werden.
|
gunicorn --bind 0.0.0.0:8080 --workers 1 main:app |
Docker Container erstellen, ausführen und deployen
Um einen Service in Cloud Run auszuführen, muss dieser in einem Docker Container vorliegen. Dazu wird zunächst ein Dockerfile im App Ordner erstellt. Diese ist einfach gehalten, es basiert auf einem Python Container, kopiert die Dateien aus dem App Ordner und definiert das Start Kommando für Gunicorn
.
In vielen Fällen sind im lokalen Entwicklungsordner Dateien vorhanden die nicht in den Container veröffentlicht werden sollten, damit Dateien explizit aus der Containererzeugung ausgeschlossen werden können kann eine .dockerignore Datei hinzugefügt werden. Diese funktioniert analog der .gitignore Dateien.
Um das Veröffentlichen auf die Artifact Registry zu vereinfachen, kann es eine gute Idee sein dem Namensschema der Registry zu folgen: location—docker.pkg.dev/your-project-id/registryname/containername:latest. Mittels docker build kann das Container Image erstellt werden:
|
docker build -t LOCATION-docker-pkg.dev/your-project-name/democontainer/democontainer:latest . |
Um das erstellt Image local zu testen, kann dieses mittels docker run
auch lokal ausgeführt werden. Wichtig ist dabei zu beachten die notwendigen Umgebungsvariablen mit zugeben und den Port zu exponieren.
|
docker run -e PORT=8080 -p 8080:8080 <image_id> |
Werden im Webservice Google Identitäten verwendet, dann müssen Informationen über den zu verwendenden Google Account mitgegeben werden. Wie dies im Detail funktioniert findet sich unter Cloud Run lokal testen.
Den ersten Container manuell deployen
Bevor es möglich ist den Cloud Run Service zu erstellen muss das Container Abbild einmal manuell in die Artifact Registry veröffentlicht werden.
|
docker push europe-west3-docker.pkg.dev/snappy-nature-350016/democontainer/democontainer:latest |
Erstellen und veröffentlichen des Container Abbilds kann auch in einem Kommando erfolgen:
|
docker buildx build -t name_of_image —push -f Dockerfile |
Cloud Run Service aufsetzen
Da der initiale Container nun in der Aritfact Registry vorhanden ist, kann der Cloud Run Service daraus erstellt werden. Für den Cloud Run Service wird eine neue Resource im main.tf
erstellt.
1 2 3 4 5 6 7 8 9 10 11 12 13 14 15 16 17 18 19 20 21 22 23 24 25 26 27 28 29 30 31 32 33 34 35 36 37 38 39 40 41 42 43
|
resource "google_cloud_run_service" "cloud_run_demo" { project = var.project_name name = var.RunJobName location = var.location template { spec { containers { image = "europe-west3-docker.pkg.dev/${var.project_name}/${var.container_name}/${var.container_name}:latest" } } } traffic { percent = 100 latest_revision = true } } # A Schedule to run the job on resource "google_cloud_scheduler_job" "timer_trigger" { name = "scheduled-cloud-run-job" project = var.project_name description = "Invoke a Cloud Run container on a schedule." schedule = "*/8 * * * *" time_zone = "Europe/Berlin" attempt_deadline = "320s" retry_config { retry_count = 2 } http_target { http_method = "POST" uri = google_cloud_run_service.cloud_run_demo.status[0].url oidc_token { service_account_email = google_service_account.cloud_shedule_caller.email } } } |
Dieser Service ist zunächst privat, alle Identitäten die diesen Service aufrufen wollen benötigen die Rolle roles/run.invoker
. Da der Cloud Scheduler den Service regelmäßig aufrufen soll, muss die Identität des Schedulers Mitglied der Rolle sein.
Nun kann der Terraform Dreiklang verwendet werden den Service und den Scheduler Job zu erstellen.
Cloud Build Trigger erstellen
Der finale Schritt um ein kontinuierliches deployment des Services zu erreichen ist einen Cloud Build Trigger einzurichten. Der Cloud Build Trigger beobachtet Veränderungen am GitHub Repository und erstellt bei jedem neuen Commit auf dem main branch eine neue Version des Cloud Run Services. Die hier vorgestellte Pipeline beinhaltet nur das Erstellen und Veröffentlichen des Containers. Für eine produktive Implementation ist unbedingt zu empfehlen auch noch ein Testschritt mit einzufügen. Mit dem folgenden Code wird der Trigger mittels Terraform erstellt:
Der Cloud Build Trigger nutzt zur Definition des Deployment Processes die Datei cloudbuild.yaml
, diese enthält die drei Schritte zum Erstellen des Container Abbilds, Veröffentlichen des Abbilds und Erzeugen einer neuen Version des Cloud Run Services.
|
steps: # build step - name: 'gcr.io/cloud-builders/docker' args: [ 'build', '-t', '$_LOCATION-docker.pkg.dev/$PROJECT_ID/$_REPO/$_IMAGE_NAME:latest', './App' ] # push to artifact registry - name: 'gcr.io/cloud-builders/docker' args: ['push', '$_LOCATION-docker.pkg.dev/$PROJECT_ID/$_REPO/$_IMAGE_NAME:latest'] # Deploy Container to Cloud Run - name: 'gcr.io/google.com/cloudsdktool/cloud-sdk' entrypoint: gcloud args: ['run', 'deploy', '$_SERVICE_NAME', '--image', '$_LOCATION-docker.pkg.dev/$PROJECT_ID/$_REPO/$_IMAGE_NAME:latest', '--region', '$_LOCATION'] images: - '$_LOCATION-docker.pkg.dev/$PROJECT_ID/$_REPO/$_IMAGE_NAME:latest' options: logging: CLOUD_LOGGING_ONLY |
- Erstellen des Container Abbilds mittels des Docker build Tools. Das erste Argument ist die Aktion
build
, das zweite und dritte beziehen sich auf den Tag des Images und das vierte gibt den Ort vor an dem nach dem Dockerfile gesucht wird
- Veröffentlichen des Container Images in die Artifact Registry mittels des Cloud Build Docker Tools. Als Argumente werden die Aktion
push
und das Ziel mitgegeben.
- Veröffentlichen der neuen Version des Images im Cloud Run Service mittels des Google Cloud SDK Tools
gcloud
Alle drei Schritte nutzen Variablen, um Projektziel, Image Tag und Ort flexibel durch den Build Trigger zu steuern. Diese Variable werden bei der Erstellung des Triggers mit definiert, d.h. dieser finden sich in der Definition des Cloud Build Triggers in der Terraform Datei. Die Variablen werden substitutions genannt, es ist zu beachten, das nutzerdefinierte Variablen mit einem Unterstrich beginnen müssen, nur Systemvariablen, wie die PROJECT_ID
.
Das Erstellen des Cloud Build Triggers kann versagen, in diesem Falle sollten die Einstellungen von Cloud Build in der Cloud Console geprüft werden. Die Service Account Berechtigungen für Cloud Run und Service Accounts müssen aktiviert sein, wie im Bild unten.
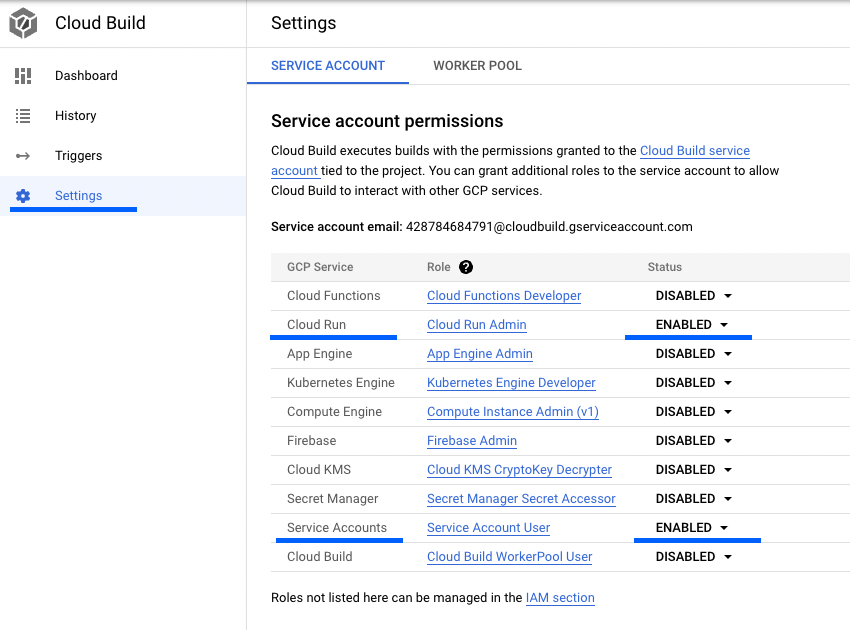
Service Account Berechtigungen für Cloud Run und Service Accounts
Zusammenfassung
Mit Hilfe von Terraform ist es möglich ein vollständig in Code definierten, kontinuierlich veröffentlichten Google Cloud Run Service zu erstellen. Dazu werden GCP Services verwendet, eine Flask Webapp in einem Container zu verpacken und diesen auf Cloud Run zu veröffentlichen.
Für Fragen erstellt gerne ein Issue oder ihr findet mich auf LinkedIn.
Der Quellcode ist in GitHub verfügbar: https://github.com/fingineering/GCPCloudRunDemo