Why do we need attribution?
Attribution – is the process of distributing the value of a purchase between the various channels, used in the funnel chain. It allows you to determine the role of each channel in profit. It is used to assess the effectiveness of campaigns, to identify more priority sources. The competent choice of the model makes it possible to optimally distribute the advertising budget. As a result, the business gets more profit and less expenses.
What models of attribution exist
The choice of the appropriate model is an important issue, because depending on the business objectives, it is better to fit something different. For example, for companies that have long been present in the industry, the priority is to know which sources contribute to the purchase. Recognition is the importance for brands entering the market. Thus, incorrect prioritization of sources may cause a decrease in efficiency. Below are the models that are widely used in the market. Each of them is guided by its own logic, it is better suited for different businesses.
First Interaction (First Click)
The value is given to the first touch. It is suitable only for several purposes and does not make it possible to evaluate the role of each component in making a purchase. It is chosen by brands who want to increase awareness and reach.
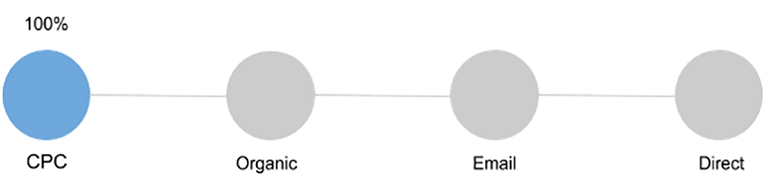
Advantages
It does not require knowledge of programming, so the introduction of a business is not difficult. A great option that effectively assesses campaigns, aimed at creating awareness and demand for new products.
Disadvantages
It limits the ability to analyze comprehensively all channels that is used to promote a brand. It gives value to the first interaction channel, ignoring the rest.
Who is suitable for?
Suitable for those who use the promotion to increase awareness, the formation of a positive image. Also allows you to find the most effective source.
Last Interaction (Last Click)
It gives value to the last channel with which the consumer interacted before making the purchase. It does not take into account the actions that the user has done up to this point, what marketing activities he encountered on the way to conversion.
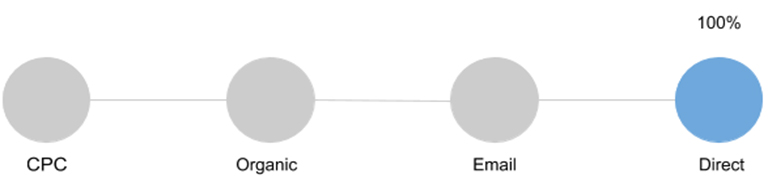
Advantages
The tool is widely used in the market, it is not difficult. It solves the problem of small advertising campaigns, where is no more than 3 sources.
Disadvantages
There is no way to track how other channels have affected the acquisition.
Who is suitable for?
It is suitable for business models that have a short purchase cycle. This may be souvenirs, seasonal offers, etc.
Last Non-Direct Click
It is the default in Google Analytics. 100% of the conversion value gives the last channel that interacted with the buyer before the conversion. However, if this source is Direct, then assumptions are counted.
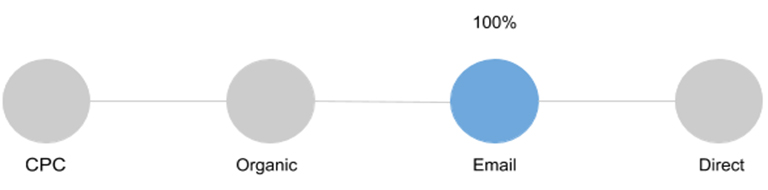
Suppose a person came from an email list, bookmarked a product, because at that time it was not possible to place an order. After a while he comes back and makes a purchase. In this case, email as a channel for attracting users would be underestimated without this model.
Who is suitable for?
It is perfect for beginners who are afraid of making a mistake in the assessment. Because it allows you to form a general idea of the effectiveness of all the involved channels.
Linear model attribution (Linear model)
The value of the conversion is divided in equal parts between all available channels.
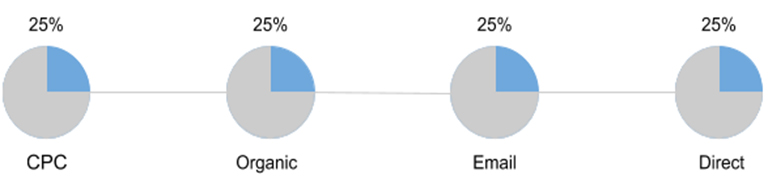
Advantages
More advanced model than previous ones, however, characterized by simplicity. It takes into account all the visits before the acquisition.
Disadvantages
Not suitable for reallocating the budget between the channels. This is due to the fact that the effectiveness of sources may differ significantly and evenly divide – it is not the best idea.
Who is suitable for?
It is performing well for businesses operating in the B2B sector, which plays a great importance to maintain contact with the customer during the entire cycle of the funnel.
Taking into account the interaction duration (Time Decay)
A special feature of the model is the distribution of the value of the purchase between the available channels by increment. Thus, the source, that is at the beginning of the chain, is given the least value, the channel at the end deserves the greatest value.
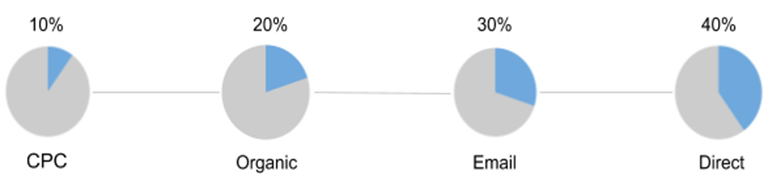
Advantages
Value is shared between all channel. The highest value is given to the source that pushed the user to make a purchase.
Disadvantages
There is no fair assessment of the effectiveness of the channels, that have made efforts to obtain the desired result.
Who is suitable for?
It is ideal for evaluating the effectiveness of advertising campaigns with a limited duration.
Position-Based or U-Shaped
40% receive 2 channels, which led the user and pushed him to purchase. 20% share among themselves the intermediate sources that participated in the chain.
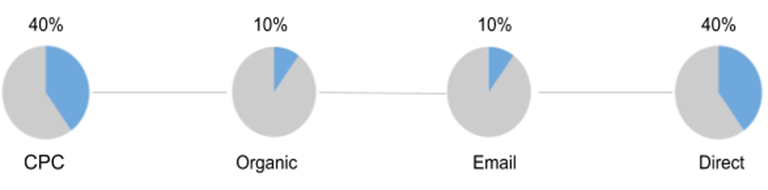
Advantages
Most of the value is divided equally between the key channels – the fact that attracted the user and closed the deal..
Disadvantages
Underestimated intermediate channels.It happens that they make it possible to more effectively promote the user chain.. Because they allow you to subscribe to the newsletter or start following the visitor for price reduction, etc.
Who is suitable for?
Interesting for businesses that focus on attracting new audiences, as well as pushing existing customers to buy.
Cons of standard attribution models
According to statistics, only 44% of foreign experts use attribution on the last interaction. Speaking about the domestic market, we can announce the numbers are much higher. However, only 18% of marketers use more complex models. There is also evidence which demonstrates that 72.4% of those who use attribution based on the last interaction, they use it not because of efficiency, but because it is simple.
What leads to a similar state of affairs?
Experts do not understand the effectiveness. Ignorance of how more complex models work leads to a lack of understanding of the real benefits for the business.
Attribution management is distributed among several employees. In view of this, different models can be used simultaneously. This approach greatly distorts the data obtained, not allowing an objective assessment of the effect of channels.
No comprehensive data storage. Information is stored in different places and does not take into account other channels. Using the analytics of the advertising office, it is impossible to work with customers in retail outlets.
You may find ways to eliminate these moments and attribution will work for the benefit of the business.
What algorithmic attribution models exist
Using one channel, there is no need to enable complex models. Attribution will be enough for the last interaction. It has everything to evaluate the effectiveness of the campaign, determine the profitability, understand the benefits for the business.
Moreover, if the number of channels increases significantly, and goals are already far beyond recognition, it will be better to give preference to more complex models. They allow you to collect all the information in one place, open up limitless monitoring capabilities, make it clear how one channel affects the other and which bundles work better together.
Below are the well-known and widely used today algorithmic attribution models.
Data-Driven Attribution
A model that allows you to track all the way that the consumer has done before making a purchase. It objectively evaluates each channel and does not take into account the position of the source in the funnel. It demonstrates how a certain interaction affected the outcome. Data-Driven attribution model is used in Google Analytics 360.
With it, you can work efficiently with channels that are underestimated in simpler models. It gives the opportunity to distribute the advertising budget correctly.
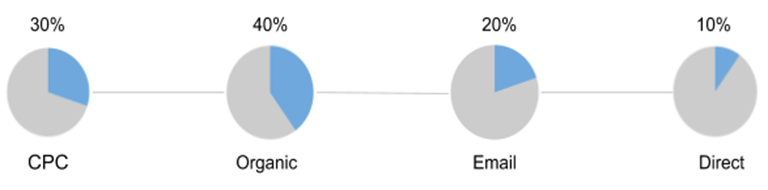
Attribution based on Markov’s Chains (Markov Chains)
Markov’s chain has been used for a long time to predict weather, matches, etc. The model allows you to find out, how the lack of a channel will affect sales. Its advantage is the ability to assess the impact of the source on the conversion, to find out which channel brings the best results.
A great option for companies that store data in one service. To implement requires knowledge of programming. It has one drawback in the form of underestimating the first channel in the chain.
OWOX BI Attribution
OWOX BI Attribution helps you assess the mutual influence of channels on encouraging a customer through the funnel and achieving a conversion.
What information can be processed:
- Upload user data from Google Analytics using flexible built-in tools.
- Process information from various advertising services.
- Integrate the model with CRM systems.
This approach makes it possible not to lose sight of any channel. Analyze the complex impact of marketing tools, correctly distributing the advertising budget.
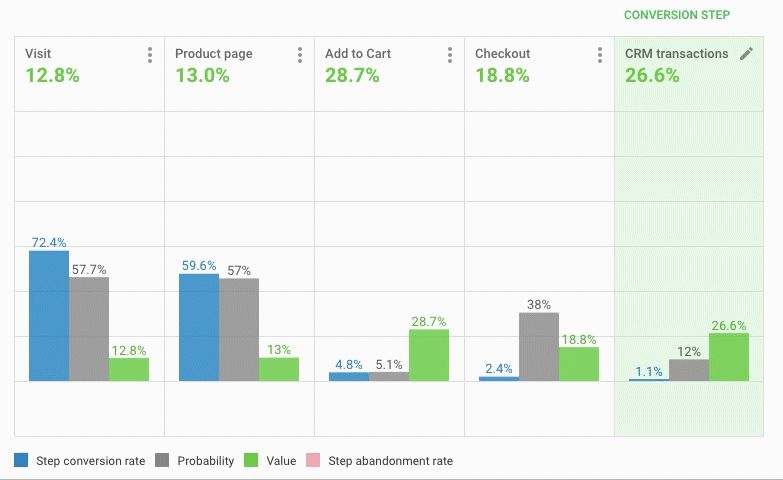
The model uses CRM information, which makes it possible to do end-to-end analytics. Each user is assigned an identifier, so no matter what device he came from, you can track the chain of actions and understand that it is him. This allows you to see the overall effect of each channel on the conversion.
Advantages
Provides an integrated approach to assessing the effectiveness of channels, allows you to identify consumers, even with different devices, view all visits. It helps to determine where the user came from, what prompted him to do so. With it, you can control the execution of orders in CRM, to estimate the margin. To evaluate in combination with other models in order to determine the highest priority advertising campaigns that bring the most profit.
Disadvantages
It is impossible to objectively evaluate the first step of the chain.
Who is suitable for?
Suitable for all businesses that aim to account for each step of the chain and the qualitative assessment of all advertising channels.
Conclusion
The above-mentioned Ad Roll study shows that 70% of marketing managers find it difficult to use the results obtained from attribution. Moreover, there will be no result without it.
To obtain a realistic assessment of the effectiveness of marketing activities, do the following:
- Determine priority KPIs.
- Appoint a person responsible for evaluating advertising campaigns.
- Define a user funnel chain.
- Keep track of all data, online and offline.
- Make a diagnosis of incoming data.
- Find the best attribution model for your business.
- Use the data to make decisions.