Der erste Artikel dieser Artikelserie Process Mining Tools beschäftigt sich mit dem Anbieter Celonis. Das 2011 in Deutschland gegründete Unternehmen ist trotz wachsender Anzahl an Wettbewerbern zum Zeitpunkt der Veröffentlichung dieses Artikels der eindeutige Marktführer im Bereich Process Mining.
Celonis Process Mining – Teil 1 der Artikelserie
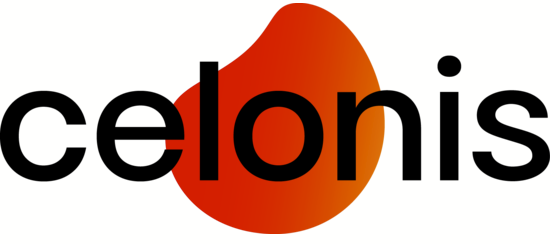
Celonis Process Mining ist 2011 als reine On-Premise-Lösung gestartet und seit 2018 auch als Cloud-Lösuung zu haben. Übersicht zu den vier verschiedenen Produktversionen der Celonis Process Mining Lösungen:
|
Celonis Snap |
Celonis Enterprise |
Celonis Academic |
Celonis Consulting |
Lizenz: |
Kostenfrei |
Kostenpflichtige Lösungspakete |
Kostenfrei |
Consulting Lizenz on Demand |
Zielgruppe: |
Für kleine Unternehmen und Einzelanwender |
Für mittel- und große Unternehmen |
Für akademische Einrichtungen und Studenten |
Für Berater |
Datenquellen: |
ServiceNow, CSV/XLS -Datei |
Beliebig (On-Premise- und Cloud – Anbindungen) |
ServiceNow, CSV/XLS/XES –Datei oder Demosysteme |
Beliebig (On-Premise- und Cloud – Anbindungen) |
Datenvolumen: |
Limitiert auf 500 MB Event-Log-Daten |
Unlimitierte Datenmengen (Größte Installation 50 TB) |
Unlimitierte Datenmengen |
Unlimitierte Datenmengen (Größte Installation 30 TB |
Architektur: |
Cloud & On-Premise |
Cloud & On-Premise |
Cloud & On-Premise |
Cloud & On-Premise |
Dieser Artikel bezieht sich im weiteren Verlauf auf die Celonis Enterprise Version, wenn nicht anders gekennzeichnet. Spezifische Unterschiede unter den einzelnen Produkten und weitere Informationen können auf der Website von Celonis entnommen werden.
Bedienbarkeit und Anpassungsfähigkeit der Analysen
In Sachen Bedienbarkeit punktet Celonis mit einem sehr übersichtlichen und einsteigerfreundlichem Userinterface. Jeder der mit BI-Tools wir z.B. „Power-BI“ oder „Tableau“ gearbeitet hat, wird sich wahrscheinlich schnell zurechtfinden.
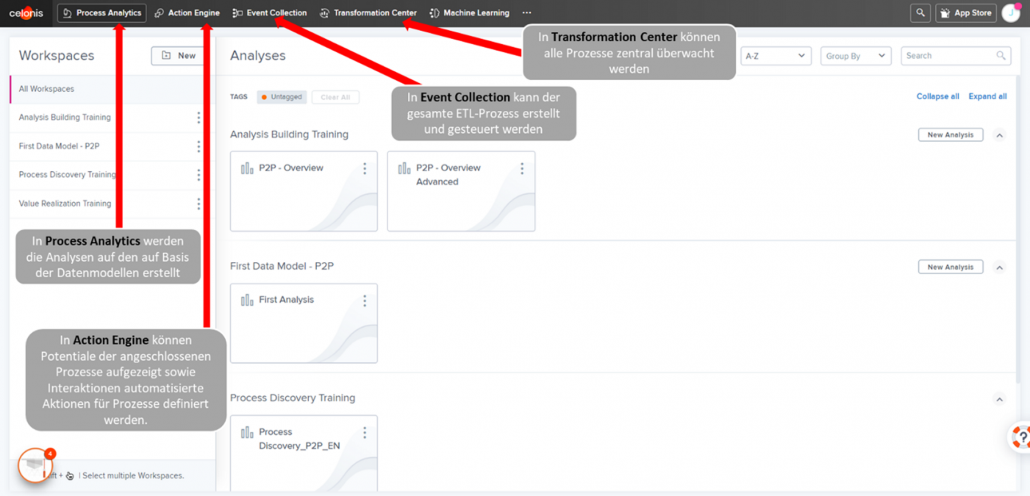
Abbildung 1: Userinterface von Celonis. Über die Reiter kann direkt von der Analyse (Process Analytics) zu den ETL-Prozessen (Event Collection) gewechselt werden.
Das Erstellen von Analysen funktioniert intuitiv und schnell, auch weil die einzelnen Komponentenbausteine lediglich per drag & drop platziert und mit den gewünschten Dimensionen und KPI’s bestückt werden müssen.
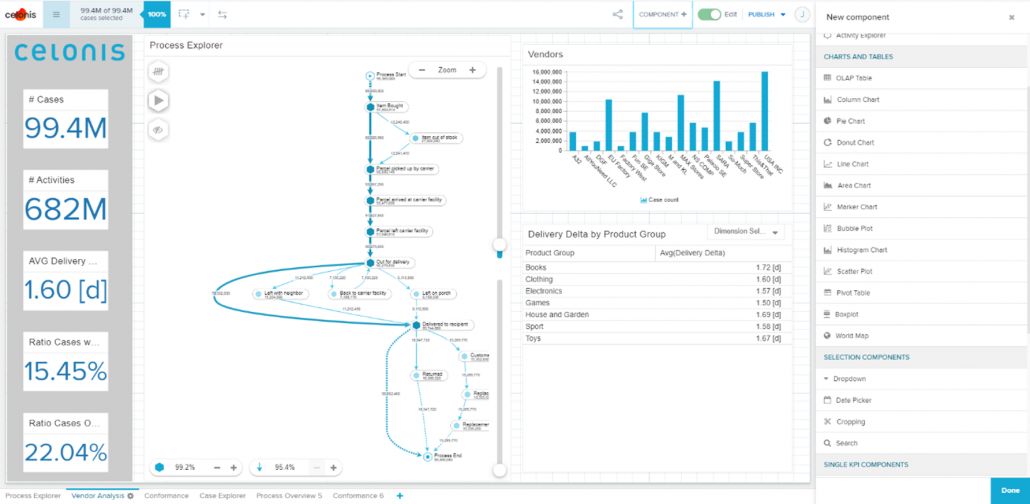
Abbildung 2: Typische Analyse im Edit Modus. Neue Komponenten können aus dem Reiter (rechts im Bild) mittels drag & drop auf der Dashboard Bearbeitungsfläche platziert werden.
Darüber hinaus bietet Celonis mit seinem kostenlosen Programm „Celonis Acadamy“ einen umfangreichen und leicht verständlichen Pool an Trainingseinheiten für die verschiedenen User-Rollen: „Snap“, „Executive“, „Business User“, „Analyst“ und „Data Engineer“. Einsteiger finden sich nach der Absolvierung der Grundkurse etwa nach vier Stunden in dem Tool zurecht.
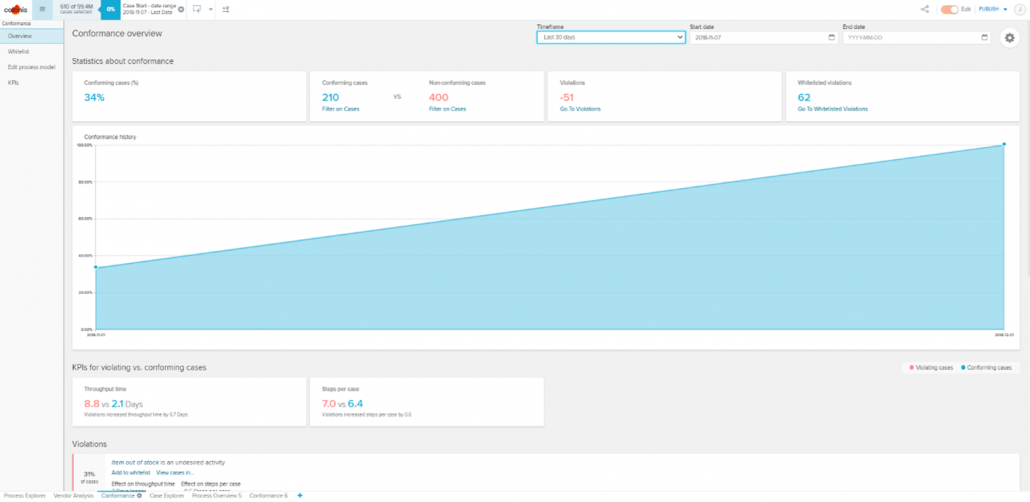
Abbildung 3: Conformance Analyse In Celonis. Es kann direkt analysiert werden, welche Art von Verstößen welche Auswirkungen haben und mit welcher Häufigkeit diese auftreten.
Die Definition von eigenen KPIs erfolgt mittels übersichtlichem Code Editor. Die verwendete proprietäre und patentierte Programmiersprache lautet PQL (Process Query Language) , dessen Syntax stark an SQL angelehnt ist und alle prozessrelevanten Berechnungen ermöglicht. Noch einsteigerfreundlicher ist der Visual Editor, in welchem KPIs alternativ mit zahlreicher visueller Unterstützung und über 130 mathematischen Operatoren erstellt werden können – ganz ohne Coding Erfahrung.
Mit Hilfe von über 30 Komponenten lassen sich alle üblichen Charts und Grafiken erstellen. Ich hatte das Gefühl, dass die Auswahl grundsätzlich ausreicht und dem Erkenntnisgewinn nicht im Weg steht. Dieses Gefühl rührt nicht zuletzt daher, dass die vorgefertigten Features, wie zum Beispiel „Conformance“ direkt und ohne Aufwand implementiert werden können und bemerkenswerte Erkenntnisse liefern. Kurzum: Ja es ist vieles vorgefertigt, aber hier wurde mit hohen Qualitätsansprüchen vorgefertigt!
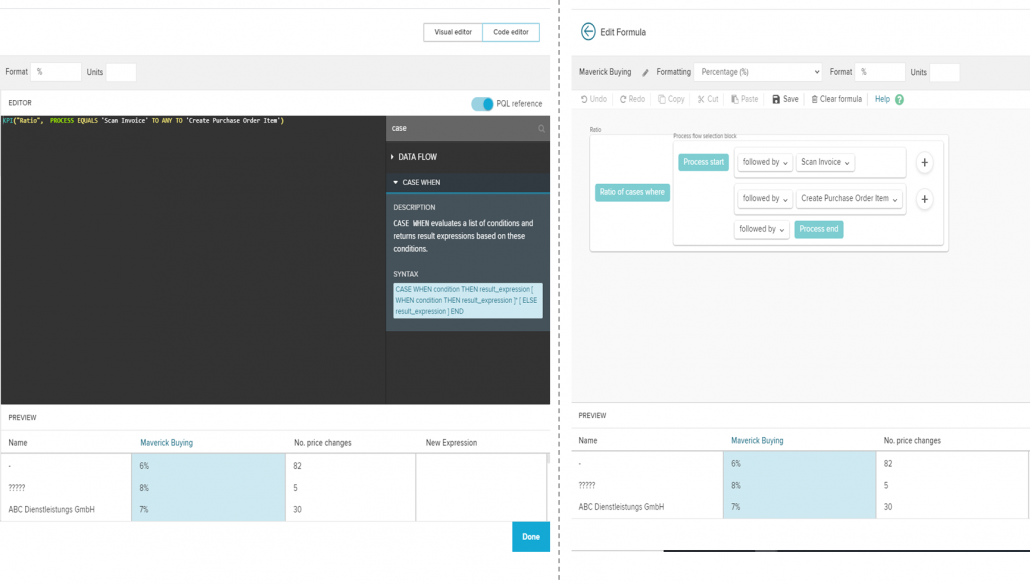
Abbildung 4: Coder Editor (links) und Visual Editor (rechts). Während im Code Editor mit PQL geschrieben werden muss, können Einsteiger im Visual Editor visuelle Hilfestellungen nehmen, um KPIs zu definieren.
Diese Flexibilität erscheint groß und bedient mehrere Zielgruppen, beginnend bei den Einsteigern. Insbesondere da das Verständnis für den Code Editor und somit für PQL durch die Arbeit mit dem Visual Code Editor gefördert wird. Wer SQL-Kenntnisse mitbringt, wird sehr schnell ohne Probleme KPIs im Code Editor definieren können. Erfahrenen Data Engineers stünde es dennoch frei, die Entwicklungsarbeit auf die Datenbankebene zu verschieben.
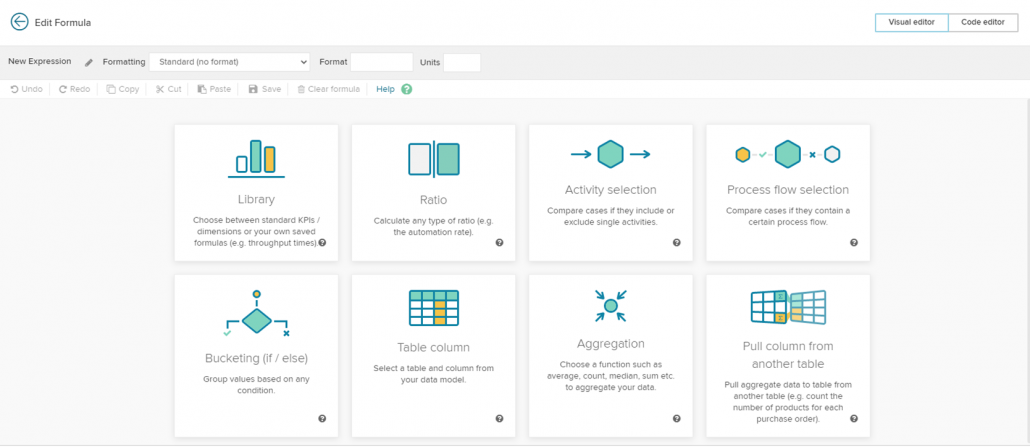
Abbildung 5: Mit Hilfe zahlreicher Möglichkeiten können Einsteiger im Visual Editor visuelle Hilfestellungen nehmen, um individuelle KPIs zu definieren.
Nachdem die ersten Analysen erstellt wurden, steht der Prozessanalyse nichts mehr im Wege. Während sich per Knopfdruck auf alle visualisierten Datenpunkte filtern lässt, unterstützt auch hier Celonis zusätzlich mit zahlreichen sogenannten ‘Auswahlansichten’, um die Entdeckung unerwünschter oder betrügerischer Prozesse so einfach wie das Googeln zu machen.
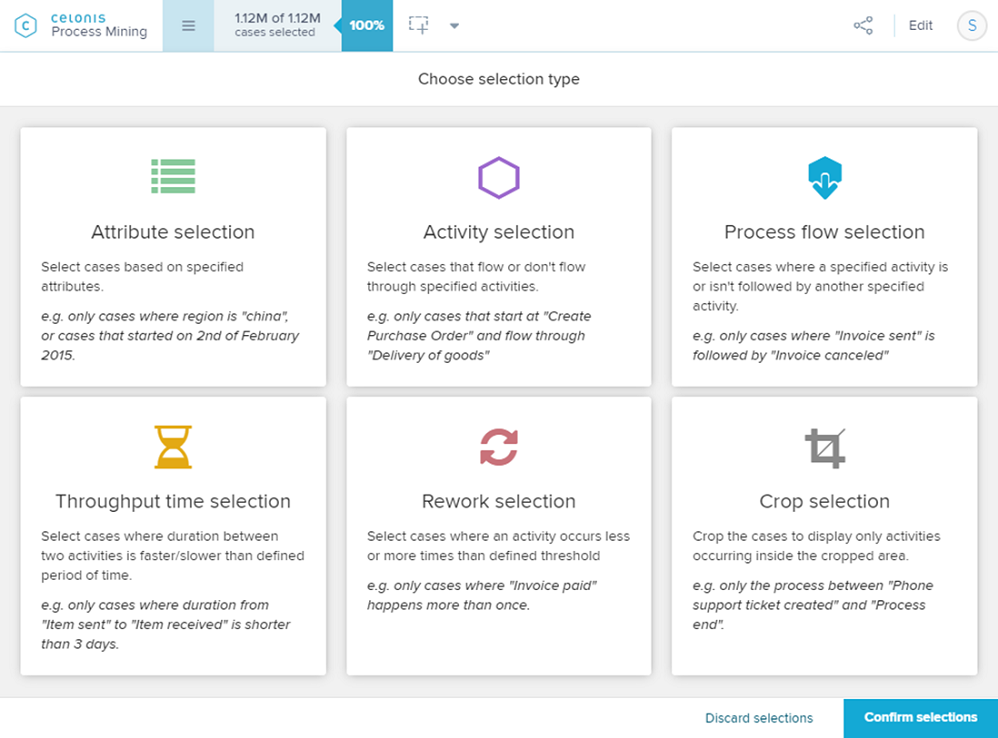
Abbildung 6: Die anwenderfreundlichen Auswahlarten ermöglichen es dem Benutzer, einfach mit wenigen Klicks nach Unregelmäßigkeiten oder Mustern in Transaktionen zu suchen und diese eingehend zu analysieren.
Integrationsfähigkeit
Die Celonis Enterprise Version ist sowohl als Cloud- und On-Premise-Lösung verfügbar. Die Cloud-Lösung bietet die folgenden Vorteile: Zum einen zusätzliche Leistungen wieCloud Connectoren, einer sogenannten Action Engine die jeden einzelnen Mitarbeiter in einem Unternehmen mit datengetriebenen nächstbesten Handlungen unterstützt, intelligenter Process Automation, Machine Learning und AI, einen App Store sowie verschiedene Boards. Diese Erweiterungen zeigen deutlich den Anspruch des Münchner Process Mining Vendors auf, neben der reinen Prozessanalyse Unternehmen beim heben der identifizierten Potentiale tatkräftig zu unterstützen. Darüber hinaus kann die Cloud-Lösung punkten mit, einer schnellen Amortisierung, bedarfsgerechter Skalierbarkeit der Kapazitäten sowie einen noch stärkeren Fokus auf Security & Compliance. Darüber hinaus erfolgen regelmäßig Updates.
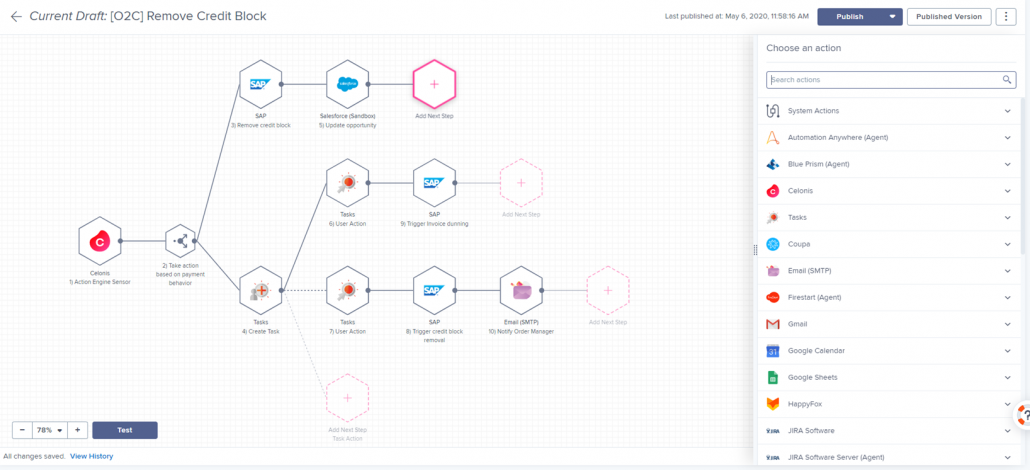
Abbildung 7: Celonis Process Automation ermöglicht Unternehmen ihre Prozesse auf intelligente Art und Weise so zu automatisieren, dass die Zielerreichung der jeweiligen Fachabteilung im Fokus stehen. Auch hier trumpft Celonis mit über 30+ vorgefertigten Möglichkeiten von der Automatisierung von Kommunikation, über Backend Automatisierung in Quellsystemen bis hin zu Einbindung von RPA Bots und vielem mehr.
Der Schwenk von Celonis scheint in Richtung Cloud zu sein und es bleibt abzuwarten, wie die On-Premise-Lösung zukünftig aussehen wird und ob sie noch angeboten wird. Je nach Ausgangssituation gilt es hier abzuwägen, welche der beiden Lösungen die meisten Vorteile bietet. In jedem Fall wird Celonis als browserbasierte Webanwendung für den Endanwender zur Verfügung gestellt. Die folgende Abbildung zeigt eine beispielhafte Celonis on-Premise-Architektur, bei welcher der User über den Webbrowser Zugang erhält.
Celonis bringt eine ausreichende Anzahl an vordefinierten Datenschnittstellen mit, wodurch sowohl gängige on-Premise Datenbanken / ERP-Systeme als auch Cloud-Dienste, wie z. B. „ServiceNow“ oder „Salesforce“ verbunden werden können. Im „App Store“ können zusätzlich sogenannte „prebuild Process-Connectors“ kostenlos erworben werden. Diese erstellen die Verbindung und erzeugen das Datenmodell (Extract and Transform) für einen Standard Prozess automatisch, so dass mit der Analyse direkt begonnen werden kann. Über 500 vordefinierte Analysen für Standard Prozesse gibt es zusätzlich im App Store. Dadurch kann die Bearbeitungszeit für ein Process-Mining Projekt erheblich verkürzt werden, vorausgesetzt das benötigte Datenmodel weicht im Kern nicht zu sehr von dem vordefinierten Model ab. Sollten Schnittstellen mal nicht vorhanden sein, können Daten auch als CSV oder XLS Format importiert werden.
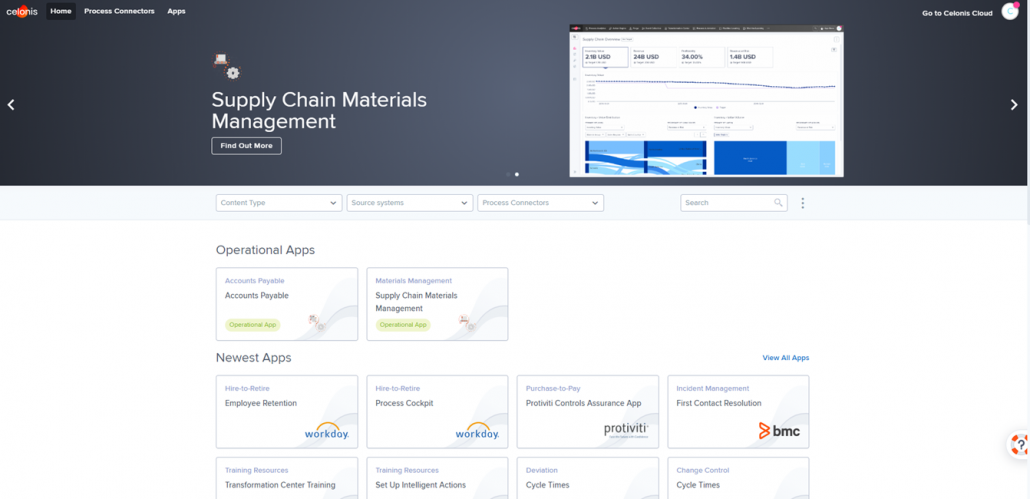
Abbildung 8: Der Celonis App Store beinhaltet über 100 Prozesskonnektoren, über 500 vorgefertigte Analysen und über 80 Action Engine Fähigkeiten die kostenlos mit der Cloud Lizenz zur Verfügung stehen
Auch wenn von einer 100%-Cloud gesprochen wird, muss für die Anbindung von unternehmensinternen on-premise Datenquellen (z. B. lokale Instanzen von SAP ERP, Oracle ERP, MS Dynamics ERP) ein sogenannter Extractor on-premise installiert werden.
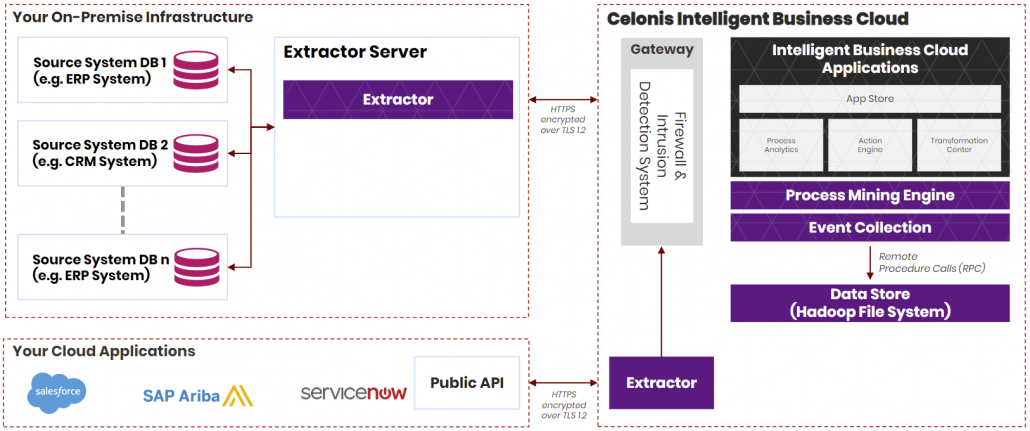
Abbildung 9: Celonis Extractor muss für die Anbindung von On-Premise Datenquellen ebenfalls On-Premise installiert werden. Dieser arbeitet wie ein Gateway zur Celonis Intelligent Business Cloud (IBC). Die IBC enthält zudem einen eigenen Extratctor für die Anbindung von Daten aus anderen Cloud-Systemen.
Celonis bietet in der Enterprise-Ausführung zudem ein umfassendes Benutzer-Berechtigungsmanagement, so dass beispielsweise für Analysen im Einkauf die Berechtigungen zwischen dem Einkaufsleiter, Einkäufern und Praktikanten im Einkauf unterschieden werden können. Auch dieser Punkt ist für viele Unternehmen eine Grundvoraussetzung für einen eventuellen unternehmensweiten Roll-Out.
Skalierbarkeit
In Punkto großen Datenmengen kann Celonis sich sehen lassen. Allein für „Uber“ verarbeitet die Cloud rund 50 Millionen Datensätze, wobei ein einzelner mehrere Terabyte (TB) groß sein kann. Der größte einzelne Datenblock, den Celonis analysiert, beträgt wohl etwas über 50 TB. Celonis bietet somit Process Mining, zeitgerecht im Bereich Big Data an und kann daher auch viele große renommierten Unternehmen zu seinen Kunden zählen, wie zum Beispiel Siemens, ABB oder BMW. Doch wie erweiterbar und flexibel sind die erstellten Datenmodelle? An diesem Punkt konnte ich keine Schwierigkeiten feststellen. Celonis bietet ein übersichtlich gestaltetes Userinterface, welches das Datenmodell mit seinen Tabellen und Beziehungen sauber darstellt. Modelliert wird mit SQL-Befehlen, wodurch eine zusätzliche Abfragesprache entfällt. Der von Celonis gewählte SQL-Dialekt ist Vertica. Dieser ist keineswegs begrenzt und bietet die ausreichende Tiefe, welche an dieser Stelle benötigt wird. Die Erweiterbarkeit sowie die Flexibilität der Datenmodelle wird somit ausschließlich von der Arbeit des Data Engineer bestimmt und in keiner Weise durch Celonis selbst eingeschränkt. Durch das Zurückgreifen auf die Abfragesprache SQL, kann bei der Modellierung auf eine sehr breite Community zurückgegriffen werden. Darüber hinaus können bestehende SQL-Skripte eingefügt und leicht angepasst werden. Und auch die Suche nach einem geeigneten Data Engineer gestaltet sich dadurch praktisch, da SQL eine der meistbeherrschten Abfragesprachen ist.
Zukunftsfähigkeit
Machine Learning umfasst Data Mining und Predictive Analytics und findet vermehrt den Einzug ins Process Mining. Auch ist es längst ein wesentlicher Bestandteil von Celonis. So basiert z. B. das Feature „Conformance“ auf Machine Learning Algorithmen, welche zu den identifizierten Prozessabweichungen den Einfluss auf das Geschäft berechnen. Aber auch Lösungen zu den Identifizierten Problemen werden von Verfahren des maschinellen Lernens dem Benutzer vorgeschlagen. Was zusätzlich in Sachen Machine Learning von Celonis noch bereitgestellt wird, ist die sogenannte Machine-Learning-Workbench, welche in die Intelligent Business Cloud integriert ist. Hier können eigene Anwendungen mit Machine Learning auf Basis der Event-Log Daten entwickelt und eingesetzt werden, um z. B. Vorhersagen zu Lieferzeiten treffen zu können.
Task Mining ist einer der nächsten Schritte im Bereich Process Mining, der den Detailgrad für Analysen von Prozessen bis hin zu einzelnen Aufgaben auf Mausklick-Ebene erhöht. Im Oktober 2019 hatte Celonis bereits angekündigt, dass die Intelligent Business Cloud um eben diese neue Technik der Datenerhebung und -analyse erweitert wird. Die beiden Methoden Prozess Analyse und Task Mining ergänzen sich ausgezeichnet. Stelle ich in der Prozess Analyse fest, dass sich eine bestimmte Aktivität besonders negativ auf meine gewünschte Performance auswirkt (z. B. Zeit), können mit Task Mining diese Aktivität genauer untersuchen und die möglichen Gründe sehr granular betrachten. So kann ich evtl. feststellen das Mitarbeiter bei einer bestimmten Art von Anfrage sehr viel Zeit in Salesforce verbringen, um Informationen zu sammeln. Hier liegt also viel Potential versteckt, um den gesamten Prozess zu verbessern. In dem z.B. die Informationsbeschaffung erleichtert wird oder evtl. der Anfragetyp optimiert wird, kann dieses Potential genutzt werden. Auch ist Task Mining die ideale Grundlage zur Formulierung von RPA-Lösungen.
Ebenfalls entscheidend für die Zukunftsfähigkeit von Process Mining ist die Möglichkeit, Verknüpfungen zwischen unterschiedlichen Geschäftsprozesse zu erkennen. Häufig sind diese untrennbar miteinander verbunden und der Output eines Prozesses bildet den Input für einen anderen. Mit prozessübergreifenden Multi-Event Logs bietet Celonis die Möglichkeit, genau diese Verbindungen aufzuzeigen. So entsteht ein einheitliches Prozessmodell für das gesamte Unternehmen. Und das unter bestimmten Voraussetzungen auch in nahezu Echtzeit.
Werden die ersten Entwicklungen im Bereich Machine Learning und Task Mining von Celonis weiter ausgebaut, ist Celonis weiterhin auf einem zukunftssicheren Weg. Unternehmen, die vor allem viel Wert auf Enterprise-Readiness und eine intensive Weiterentwicklung legen, dürften mit Celonis auf der sicheren Seite sein.
Preisgestaltung
Die Preisgestaltung der Enterprise Version wird von Celonis nicht transparent kommuniziert. Angeboten werden verschiedene kostenpflichtige Lösungspakete, welche sich aus den Anforderungen eines Projektes ergeben. Generell stufe ich die Celonis Enterprise Version als Premium Produkt ein. Dies liegt auch daran, weil die Basisausführung der Celonis Enterprise Version bereits sehr umfänglich ist und neben der Software Subscription standardmäßig auch mit Wartung und Support kommt. Zusätzlich steckt mittlerweile sehr viel Entwicklungsarbeit in der Celonis Process Mining Plattform, welche weit über klassische Process Discovery Solutions hinausgeht. Für kleinere Unternehmen mit begrenztem Budget gibt es daher zwischen der kostenfreien Snap Version und den Basis Paketen der Enterprise Version oft keine Interimslösung.
Fazit
Insgesamt stellt Celonis ein unabhängiges und leistungsstarkes Process Mining Tool in der Cloud bereit. Gehört die Cloud zur Unternehmensstrategie, ist man bei Celonis an der richtigen Adresse. Die „prebuild Process-Connectors“ und die vordefinierten Analysen können ein Process Mining Projekt signifikant beschleunigen und somit die Time-to-Value lukrativ verkürzen. Die Analyse Tools sind leicht bedienbar und schaffen dank integrierter Machine Learning Algorithmen Optimierungspotentiale. Positiv ist auch zu bewerten, dass Celonis ohne speziellen Syntax auskommt und mittelmäßige SQL-Fähigkeiten somit völlig ausreichend sind, um Prozessanalysen vollumfänglich durchzuführen. Diesen vielen positiven Aspekten steht eigentlich nur die hohe Preisgestaltung für die Enterprise Version gegenüber. Ob diese im Einzelfall gerechtfertigt ist, sollte situationsabhängig evaluiert werden. Sicherlich richtet sich Celonis Enterprise in erster Linie an größere Unternehmen, welche komplexe Prozesse mit hohen Datenvolumina analysieren möchte. Mit Celonis-Snap können jedoch auch kleine Unternehmen und Start-ups einen begrenzten Einblick in dieses gut gelungene Process Mining Tool erhalten.