In 2009, Google Chief Economist Hal Varian said to the McKinsey Quarterly that “the sexy job in the next 10 years will be statisticians.”
At the time, it was hard to believe. But more than a decade later, we can’t get around the importance of data. Where once oil ruled the world, data is now catching up—quickly. That calls for more and better data scientists. In this article, we’ll explain to you how to find them.
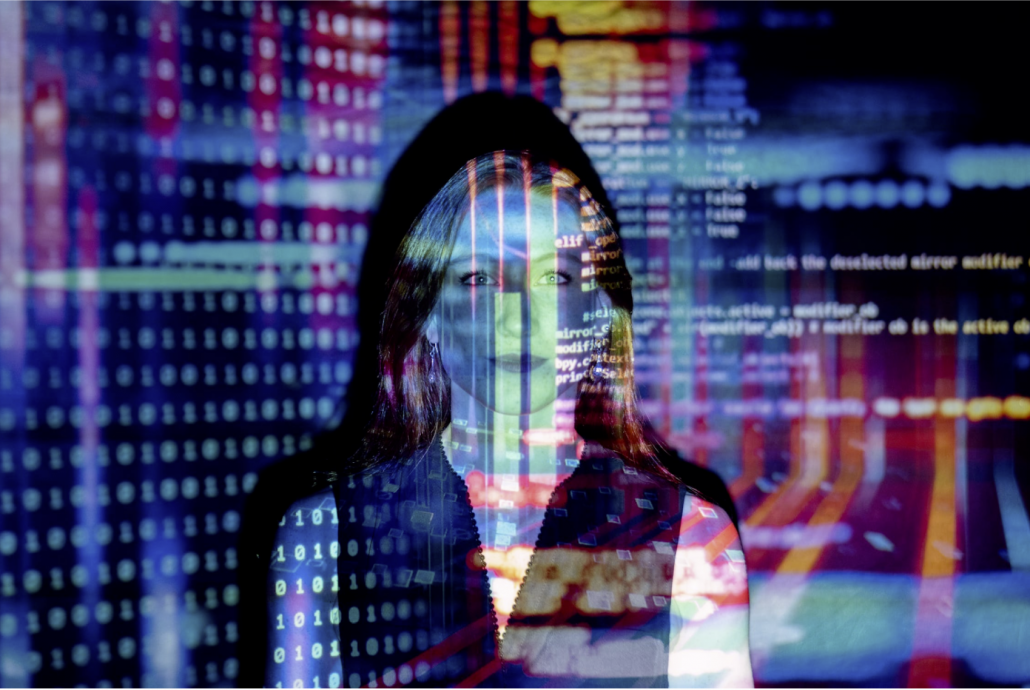
Source: https://www.pexels.com/
Why is it so hard to find good data scientists?
The demand for data scientist roles has increased by 650 percent since 2012, and that number will continue to grow as the amount of data—and power it holds—grows steadily, too.
But unsurprisingly, there hasn’t been an increase of 650 percent in available data scientists on the job market. Even though the job is a lot sexier—and better paid—than ten years ago, many employers are still struggling to fill their empty seats with talented data scientists.
McKinsey predicted that there would be a shortage of between 140,000 and 190,000 people with analytical skills in the U.S. alone in 2018, and even in 2022 good data scientists, data analysts, forecasting analysts, modelling analysts, machine learning scientists, are hard to find.
Add to that another 1.5 million managers who will also need to at least understand how data analysis drives decision-making, and you can see how employers can be in a bit of a pickle.
Why thoroughly screening data scientists is still crucial
Even though demand is growing much faster than the number of data scientists, companies can’t simply settle for the first data lover who’s available from Monday to Friday.
It’s no longer the company with the most data that wins the game. The ones who are taking the lead are the ones that are able to get the most out of data. They can pull valuable information that helps with decision-making and innovation out of even the smallest pieces of data—and they’re right, over and over again.
This is why it’s vital to check if applicants have the skills you need to derive valuable input out of data. You’ll be basing a lot of business decisions on what these data scientists tell you, so best make sure they’re right.
But what makes someone a great data scientist? Some people turn their life around and go from being a maths teacher to following a 12-week data science boot camp or online data science course and quickly get the hang of it—others are top of their class, but aren’t confident enough data scientists to inform your business on its next big move.
The truth is that the skills a valuable data scientist has, will have to develop over the years. It’s not just the data literacy, hard skills and the brain for maths—they’ll also need to be able to present and communicate their findings the right way.
Finding the right data scientists using a data science job assessment
So, you’ll want to choose your data scientists carefully, but how do you do that? Resumes and portfolios might seem impressive, but how do you actually find out if someone has the skills you’re looking for—especially if you don’t have anyone on board yet that knows what to ask?
The easiest and most effective thing to do, is to screen candidates early in the process, using a data science test that’s been created by a real-life expert.
This will ensure that relevant questions are being asked, and you get a clear idea of who’s worth going through the hiring process with—and who isn’t.
In this article, we’ll walk you through four steps that will help you set up a data science job assessment that is of real value to your hiring managers. Let’s get started.
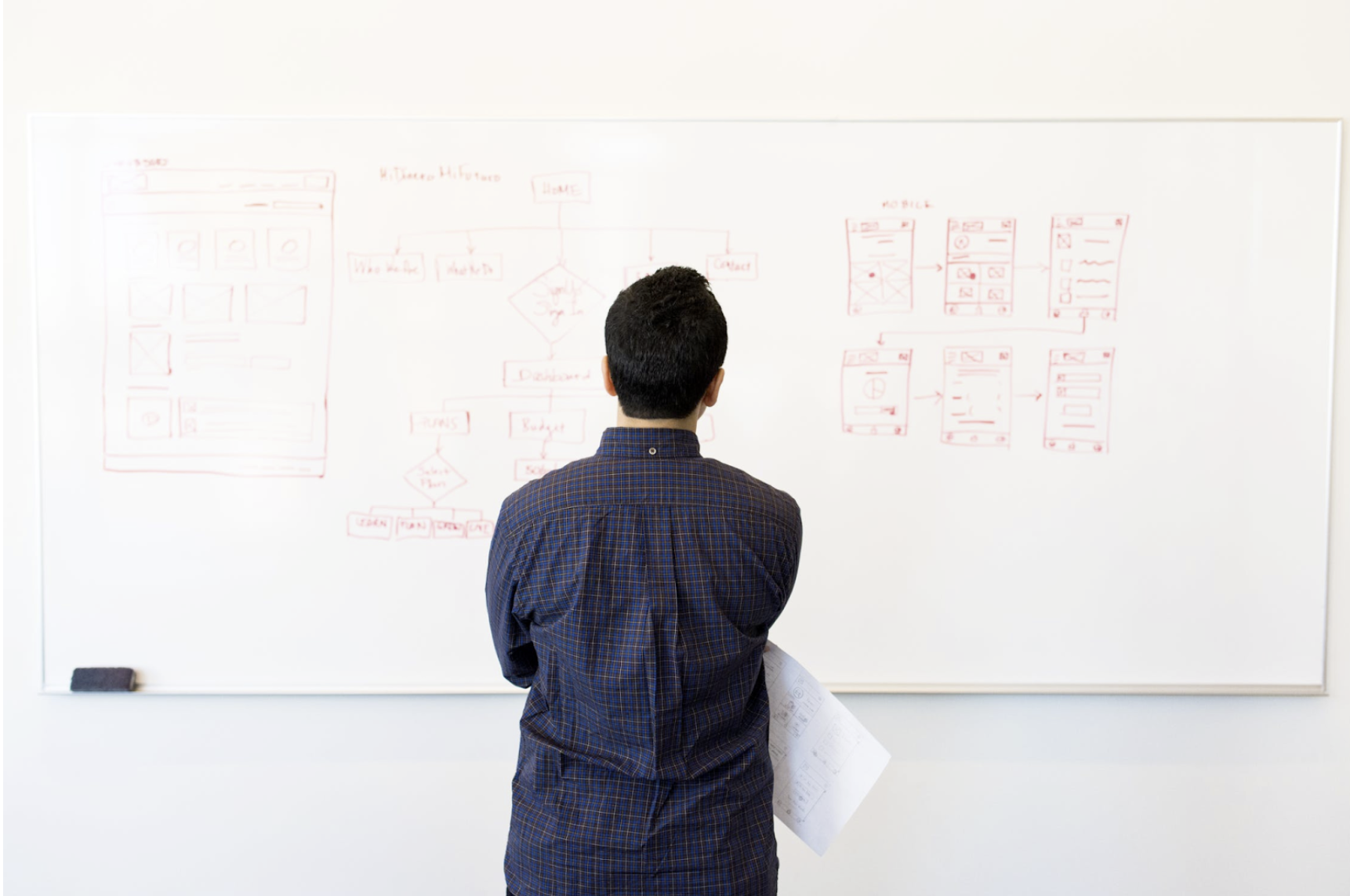
Source: https://www.pexels.com/
Step 1: Choose the right platform
You could, of course, draw up an online survey and create a test in there to send out to all applicants, but these might be hard to ‘grade’—although you’ll develop a tremendous respect for teachers along the way.
In many cases, it’s better to choose a dedicated platform that has tests available, and will help you swift through the results effortlessly.
Before you start looking for platforms, make a list of absolute needs that you won’t compromise on. Ask yourself at least the following questions:
- What types of tests are you looking for? Only hard skills, or also soft skills? If you need both, look for a platform that offers both—mixing and matching can be time-consuming.
- Will there be tests readily available, or are you looking for a platform that allows you to create your own tests?
- Does the platform have experience with companies like yours?
- How are the tests presented to candidates, and how do you want the test results presented to your hiring managers?
- And last but not least: what are you willing to spend on a job assessment platform? Do they charge per candidate, a flat fee, or would you prefer an annual subscription?
Once you’ve chosen a platform that is right for you, the fun can begin.
Step 2: Start with a hard skills assessment
For roles like data scientists, you’ll be initially focusing on whether they possess the right hard skills. Depending on the specific role, you can test core data science topics such as:
Statistics
You’re expecting your future data scientist to be fluent in statistics. Depending on the level you’re hiring at, you might want to throw in a few questions that quickly test how fast someone can see through the woods in a mess of statistics, and if they can interpret them the right way.
Machine learning
For some more senior roles, machine learning is becoming increasingly important in the world of data science. If this is the case for the role you’re hiring for, test to see if someone knows how to use data to feed it to machine learning and build awesome products.
Neural networks
A big part of data science is knowing how to work with neural networks. Neural networks are a way to solve problems through trial and error, based on human and animal brains. It’s incredibly helpful if your data scientist’s brain can use them.
Deep learning
Deep learning is a subfield of machine learning that can be necessary in specific data science roles. It works more closely to the way the human brain makes decisions, so this will require a specific set of test questions.
Collecting data
All that data has to come from somewhere, right? Your data scientists should not only be able to read and process data, but also know where and how to get the most valuable input. For this, include some questions about data extraction, data transformation, and data loading. This can also include tests on Excel and querying languages like SQL.
Storing data
Databases should look nothing like the average teenage bedroom. Meaning that they should be nice and tidy, making it easier to extract valuable information from them. Since data isn’t just numbers, but can be anything from video to reviews, it’s crucial that you hire a data scientist who knows how to store this correctly.
Analyzing and modeling data
Data wrangling, data exploration, analysis, and modeling need in-depth understanding of math and programming, but luckily, even data scientists get some help.
Data scientists use analytical tools like Apache Spark, D3.js Python, and many, many more to analyze all that data. If you’re using a specific one in your company and want your data scientists to be able to hit the ground running, quickly test if they’re actually able to use the tools they list on their resume.
Visualizing and presenting data
At the end of the day, data scientists will have to be able to communicate their findings to other departments with people who are less data-savvy. For this, they often use tools that help them visualize data to explain it in a more easy-to-grasp way.
Test if your next data scientist is able to do that with a quick check on their skills in tools like Tableau, PowerBI, Plotly, Bokeh, or whichever one you use.
Step 3: Continue with a soft skill assessment
Your friendly neighborhood data scientist should not only be a math genius, they should possess the right soft skills too. If they’re impossible to work with, you won’t reap the benefits of their skill set. Productivity will suffer, and team morale might also take a hit. Here are some soft skills to test your candidates on:
- Business-oriented: ultimately, your data scientist will be fueling your decision-making process. This means they’ll have to have a good head for business, on top of simply understanding the numbers.
- Communication skills: sure, everyone in your company preferably has some of these, but since data scientists play such an important role in decision-making, you’ll want them to be able to express themselves well—and listen to what you’re asking from them.
- Teamwork: your data scientists shouldn’t be on a little island somewhere in the company. The more they integrate with other departments, the easier it is for them to determine what your business needs from them.
- Critical thinking skills: this one’s pretty self-explanatory, but the more critical your data scientist, the more reassurance you’ll have that data is correctly interpreted.
- Creativity: data is less dry than it seems. From data storage to finding connections and problem-solving: it all requires some form of creative thinking.
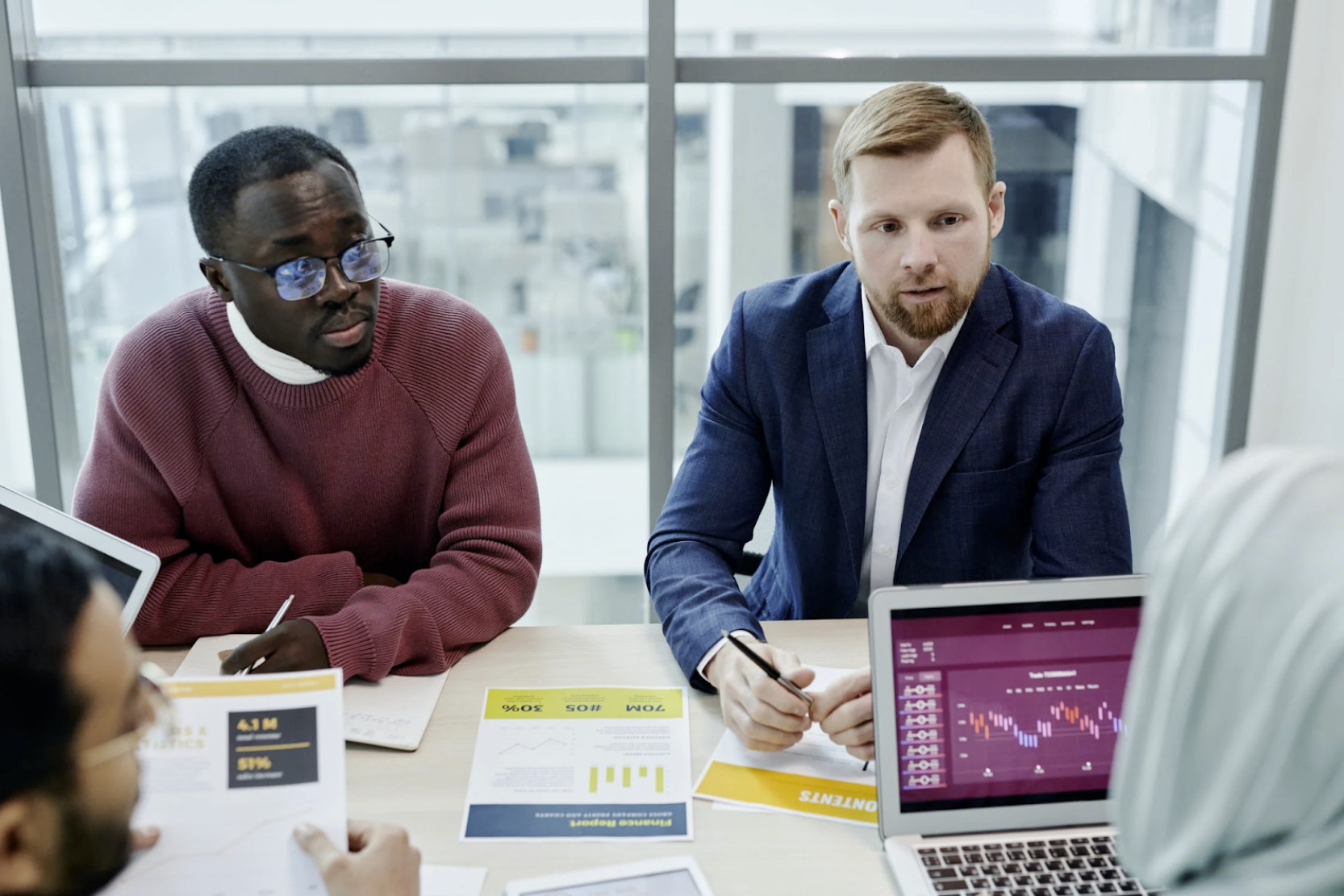
Source: https://www.pexels.com/
Step 4: Follow up on the test results
If you want to make the most of your data science job assessment, it shouldn’t just be a test to see who goes through to the next round. For the candidates that ‘pass’, you can customize the questions in their follow-up interview based on the strengths and weaknesses they showed in their test.
Because the test they took says a lot, but at the same time—it’s just a snapshot. Did they score remarkably high on certain skills? Ask them how they got to be so experienced in that, and what projects contributed most to that.
Did you notice that they struggled with questions about X? Ask how they are planning to improve on that and how they make sure this doesn’t impact the quality of their work for the time being—are they calling in help from a peer, or do they simply take more time to figure things out?
These types of follow-up questions steer a job interview in a much more real-life direction: it’s not a generic set of questions that any company could ask any employee, but a real conversation between you and the candidate, in which you can evaluate if they fit in the future of the company—and if your company fits in theirs.
Ready to start the hiring process?
With these tips, we’re sure you’ll get some extra reassurance that your next hire will be a great fit—not just based on their previous experience and a couple of interviews. If you want, you can keep reading about data science jobs—or simply start hiring. Good luck!