Step-by-Step:
Neue Möglichkeiten zur Aufdeckung von Compliance-Verstößen mit Process Analytics
Im Zuge der fortschreitenden Digitalisierung findet derzeit ein enormer Umbruch der alltäglichen Arbeit hin zur lückenlosen Erfassung aller Arbeitsschritte in IT-Systemen statt. Darüber hinaus sehen sich Unternehmen mit zunehmend verschärften Regulierungsanforderungen an ihre IT-Systeme konfrontiert.
Der unaufhaltsame Trend hin zur vernetzten Welt („Internet of Things“) wird die Möglichkeiten der Prozesstransparenz noch weiter vergrößern – jedoch werden bereits jetzt viele Prozesse im Unternehmensbereich über ein oder mehrere IT-Systeme erfasst. Jeder Mitarbeiter, aber auch jeder automatisiert ablaufende Prozess hinterlässt viele Datenspuren in IT-Backend-Systemen, aus denen Prozesse rückwirkend oder in Echtzeit nachgebildet werden können. Diese umfassen sowohl offensichtliche Prozesse, wie etwa den Eintrag einer erfassten Bestellung oder Rechnung, als auch teilweise verborgene Prozesse, wie beispielsweise die Änderung bestimmter Einträge oder Löschung dieser Geschäftsobjekte.
Read this article in English:
“Process Analytics – Data Analysis for Process Audit & Improvement”
1 Das Verständnis von Process Analytics
Process Analytics ist eine datengetriebene Methodik der Ist-Prozessanalyse, die ihren Ursprung in der Forensik hat. Im Kern des dieser am Zweck orientierten Analyse steht das sogenannte Process Mining, eine auf die Rekonstruktion von Prozessen ausgerichtetes Data Mining. Im Zuge der steigenden Bedeutung der Computerkriminalität wurde es notwendig, die Datenspuren, die potenzielle Kriminelle in IT-Systemen hinterließen, zu identifizieren und zu analysieren, um das Geschehen so gut wie möglich zu rekonstruieren.
Mit dem Trend hin zu Big Data Analytics hat Process Analytics nicht nur neue Datengrundlagen erhalten, sondern ist als Analysemethode weiterentwickelt worden. Zudem ermöglicht die Visualisierung dem Analysten oder Berichtsempfänger ein tief gehendes Verständnis auch komplexerer Geschäftsprozesse.
Während in der konventionellen Prozessanalyse vor allem Mitarbeiterinterviews und Beobachtung der Mitarbeiter am Schreibtisch durchgeführt werden, um tatsächlich gelebte Prozesse zu ermitteln, ist Process Analytics eine führende Methode, die rein faktenbasiert und damit objektiv an die Prozesse herangeht. Befragt werden nicht die Mitarbeiter, sondern die IT-Systeme, die nicht nur alle erfassten Geschäftsobjekte tabellenorientiert abspeichern, sondern auch im Hintergrund – unsichtbar für die Anwender – jegliche Änderungsvorgänge z. B. an Bestellungen, Rechnungen oder Kundenaufträgen lückenlos mit einem Zeitstempel (oft Sekunden- oder Millisekunden-genau) protokollieren.
2 Die richtige Auswahl der zu betrachtenden Prozesse
Heute arbeitet nahezu jedes Unternehmen mit mindestens einem ERP-System. Da häufig noch weitere Systeme eingesetzt werden, lässt sich klar herausstellen, welche Prozesse nicht analysiert werden können: Solche Prozesse, die noch ausschließlich auf Papier und im Kopf der Mitarbeiter ablaufen, also typische Entscheiderprozesse auf oberster, strategischer Ebene, die nicht in IT-Systemen erfasst und dementsprechend nicht ausgewertet werden können. Operative Prozesse werden hingegen in der Regel nahezu lückenlos in IT-Systemen erfasst und operative Entscheidungen protokolliert.
Zu den operativen Prozessen, die mit Process Analytics sehr gut rekonstruiert und analysiert werden können und gleichermaßen aus Compliance-Sicht von höchstem Interesse sind, gehören beispielsweise Prozesse der:
- Beschaffung
- Logistik / Transport
- Vertriebs-/Auftragsvorgänge
- Gewährleistungsabwicklung
- Schadensregulierung
- Kreditgewährung
Process Analytics bzw. Process Mining ermöglicht unabhängig von der Branche und dem Fachbereich die größtmögliche Transparenz über alle operativen Geschäftsprozesse. Für die Audit-Analyse ist dabei zu beachten, dass jeder Prozess separat betrachtet werden sollte, denn die Rekonstruktion erfolgt anhand von Vorgangsnummern, die je nach Prozess unterschiedlich sein können. Typische Vorgangsnummern sind beispielsweise Bestell-, Auftrags-, Kunden- oder Materialnummern.
3 Auswahl der relevanten IT-Systeme
Grundsätzlich sollte jedes im Unternehmen eingesetzte IT-System hinsichtlich der Relevanz für den zu analysierenden Prozess untersucht werden. Für die Analyse der Einkaufsprozesse ist in der Regel nur das ERP-System (z. B. SAP ERP) von Bedeutung. Einige Unternehmen verfügen jedoch über ein separates System der Buchhaltung (z.B. DATEV) oder ein CRM/SRM (z. B. von Microsoft), die dann ebenfalls einzubeziehen sind.
Bei anderen Prozessen können außer dem ERP-/CRM-System auch Daten aus anderen IT-Systemen eine entscheidende Rolle spielen. Gelegentlich sollten auch externe Daten integriert werden, wenn diese aus extern gelagerten Datenquellen wichtige Prozessinformationen liefern – beispielsweise Daten aus der Logistik.
4 Datenaufbereitung
Vor der datengetriebenen Prozessanalyse müssen die Daten, die auf Prozessaktivitäten direkt oder indirekt hindeuten, in den Datenquellen identifiziert, extrahiert und aufbereitet werden. Die Daten liegen in Datenbanktabellen und Server-Logs vor und werden über ein Data Warehousing Verfahren zusammengeführt und in ein Prozessprotokoll (unter den Process Minern i.d.R. als Event Log bezeichnet) umformuliert.
Das Prozessprotokoll ist in der Regel eine sehr große und breite Tabelle, die neben den eigentlichen Prozessaktivitäten auch Parameter enthält, über die sich Prozesse filtern lassen, beispielsweise Informationen über Produktgruppen, Preise, Mengen, Volumen, Fachbereiche oder Mitarbeitergruppen.
5 Prüfungsdurchführung
Die eigentliche Prüfung erfolgt visuell und somit intuitiv vor einem Prozessflussdiagramm, das die tatsächlichen Prozesse so darstellt, wie sie aus den IT-Systemen extrahiert werden konnten.
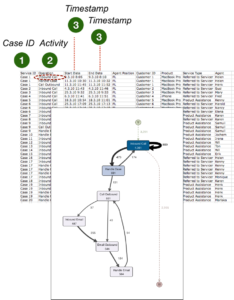
Process Mining – Beispielhafter Process Flow mit Fluxicon Disco (www.fluxicon.com)
Das durch die Datenaufbereitung erstellte Prozessprotokoll wird in eine Datenvisualisierungssoftware geladen, die dieses Protokoll über die Vorgangsnummern und Zeitstempel in einem grafischen Prozessnetzwerk darstellt. Die Prozessflüsse werden also nicht modelliert, wie es bei den Soll-Prozessen der Fall ist, sondern es „sprechen“ die IT-Systeme.
Die Prozessflüsse werden visuell dargestellt und statistisch ausgewertet, so dass konkrete Aussagen über die im Hinblick auf Compliance relevante Prozess-Performance und -Risiken getroffen werden können.
6 Abweichung von Soll-Prozessen
Die Möglichkeit des intuitiven Filterns der Prozessdarstellung ermöglicht auch die gezielte Analyse von Ist-Prozessen, die von den Soll-Prozessverläufen abweichen.
Die Abweichung der Ist-Prozesse von den Soll-Prozessen wird in der Regel selbst von IT-affinen Führungskräften unterschätzt – mit Process Analytics lassen sich nun alle Abweichungen und die generelle Prozesskomplexität auf ihren Daten basierend untersuchen.
6 Erkennung von Prozesskontrollverletzungen
Die Implementierung von Prozesskontrollen sind Bestandteil eines professionellen Internen Kontrollsystems (IKS), die tatsächliche Einhaltung dieser Kontrollen in der Praxis ist jedoch häufig nicht untersucht oder belegt. Process Analytics ermöglicht hier die Umgehung des Vier-Augen-Prinzips bzw. die Aufdeckung von Funktionstrennungskonflikten. Zudem werden auch die bewusste Außerkraftsetzung von internen Kontrollmechanismen durch leitende Mitarbeiter oder die falsche Konfiguration der IT-Systeme deutlich sichtbar.
7 Erkennung von bisher unbekannten Verhaltensmustern
Nach der Prüfung der Einhaltung bestehender Kontrollen, also bekannter Muster, wird Process Analytics weiterhin zur Neuerkennung von bislang unbekannten Mustern in Prozessnetzwerken, die auf Risiken oder gar konkrete Betrugsfälle hindeuten und aufgrund ihrer bisherigen Unbekanntheit von keiner Kontrolle erfasst werden, genutzt. Insbesondere durch die – wie bereits erwähnt – häufig unterschätzte Komplexität der alltäglichen Prozessverflechtung fallen erst durch diese Analyse Fraud-Szenarien auf, die vorher nicht denkbar gewesen wären. An dieser Stelle erweitert sich die Vorgehensweise des Process Mining um die Methoden des maschinellen Lernens (Machine Learning), typischerweise unter Einsatz von Clustering, Klassifikation und Regression.
8 Berichterstattung – auch in Echtzeit möglich
Als hocheffektive Audit-Analyse ist Process Analytics bereits als iterative Prüfung in Abständen von drei bis zwölf Monaten ausreichend. Nach der erstmaligen Durchführung werden bereits Compliance-Verstöße, schwache oder gar unwirksame Kontrollen und gegebenenfalls sogar Betrugsfälle zuverlässig erkannt. Die Erkenntnisse können im Nachgang dazu genutzt werden, um die Schwachstellen abzustellen. Eine weitere Durchführung der Analyse nach einer Karenzzeit ermöglicht dann die Beurteilung der Wirksamkeit getroffener Maßnahmen.
In einigen Anwendungsszenarien ist auch die nahtlose Anbindung der Prozessanalyse mit visuellem Dashboard an die IT-Systemlandschaft zu empfehlen, so dass Prozesse in nahezu Echtzeit abgebildet werden können. Diese Anbindung kann zudem um Benachrichtigungssysteme ergänzt werden, so dass Entscheider und Revisoren via SMS oder E-Mail automatisiert über aktuellste Prozessverstöße informiert werden. Process Analytics wird somit zum Realtime Analytics.
Fazit
Process Analytics ist im Zuge der Digitalisieurng die hocheffektive Methodik aus dem Bereich der Big Data Analyse zur Aufdeckung Compliance-relevanter Tatbestände im gesamten Unternehmensbereich und auch eine visuelle Unterstützung bei der forensischen Datenanalyse.