Den Auftakt dieser Artikelserie zum Vergleich von BI-Tools macht die Softwarelösung Power BI von Microsoft. Solltet ihr gerade erst eingestiegen sein, dann schaut euch ruhig vorher einmal die einführenden Worte und die Ausführungen zur Datenbasis an.
Lizenzmodell
Power BI ist in seinem Kern ein Cloud-Dienst und so ist auch die Ausrichtung des Lizenzmodells. Der Bezug als Stand-Alone SaaS ist genauso gut möglich, wie auch die Nutzung von Power BI im Rahmen des Serviceportfolios Office 365 von Microsoft. Zusätzlich besteht aber auch die Möglichkeit die Software lokal, also on premise laufen zu lassen. Beachten sollten man aber die eingeschränkte Funktionalität gegenüber der cloudbasierten Alternative.
Power BI Desktop, das Kernelement des Produktportfolios, ist eine frei verfügbare Anwendung. Damit schafft Microsoft eine geringe Einstiegsbarriere zur Nutzung der Software. Natürlich gibt es, wie auf dem Markt üblich, Nutzungsbeschränkungen, welche den User zum Kauf animieren. Interessanterweise liegen diese Limitierungen nicht in den wesentlichen Funktionen der Software selbst, also nicht im Aufbau von Visualisierungen, sondern vor allem in der beschränkten Möglichkeit Dashboards in einem Netzwerk zu teilen. Beschränkt auch deshalb, weil in der freien Version ebenfalls die Möglichkeit besteht, die Dashboards teilen zu können, indem eine Datei gespeichert und weiter versendet werden kann. Microsoft rät natürlich davon ab und verweist auf die Vorteile der Power BI Pro Lizenz. Dem ist i.d.R. zuzustimmen, da (wie im ersten Artikel näher erläutert) ein funktionierendes Konzept zur Data Governance die lokale Erstellung von Dashboards und manuelle Verteilung nicht erlauben würde. Sicherlich gibt es Firmen die Lizenzkosten einsparen wollen und funktionierende Prozesse eingeführt haben, um eine Aktualität und Korrektheit der Dashboards zu gewährleisten. Ein Restrisiko bleibt! Demgegenüber stehen relativ geringe Lizenzkosten mit $9,99 pro Monat/User für eine Power BI Pro Lizenz, nutzt man die cloud-basierte Variante mit dem Namen Power BI Service. Das Lizenzmodell ist für den Einstieg mit wenigen Lizenzen transparent gestaltet und zudem besteht keine Verpflichtung zur Abnahme einer Mindestmenge an Lizenzen, also ist der Einstieg auch für kleine Unternehmen gut möglich. Das Lizenzmodell wird komplexer bei intensivierter Nutzung der Cloud (Power BI Service) und dem zeitgleichen Wunsch, leistungsfähige Abfragen durchzuführen und große Datenmengen zu sichern. Mit einer Erweiterung der Pro Lizenz auf die Power BI Premium Lizenz, kann der Bedarf nach höheren Leistungsanforderungen gedeckt werden. Natürlich sind mit diesem Upgrade Kapazitätsgrenzen nicht aufgehoben und die Premium Lizenz kann je nach Leistungsanforderungen unterschiedliche Ausprägungen annehmen und Kosten verursachen. Microsoft hat sogenannte SKU´s definiert, welche hier aufgeführt sind. Ein Kostenrechner steht für eine Kostenschätzung online bereit, wobei je nach Anforderung unterschiedliche Parameter zu SKU`s (Premium P1, P2, P3) und die Anzahl der Pro Lizenzen wesentliche Abweichungen zum kalkulierten Preis verursachen kann. Die Kosten für die Premium P1 Lizenz belaufen sich auf derzeit $4.995 pro Monat und pro Speicherressource (Cloud), also i.d.R. je Kunde. Sollte eine cloud-basierte Lösung aus Kosten, technischen oder sogar Data Governance Gründen nicht möglich sein, kann der Power BI Report Server auf einer selbst gewählten Infrastruktur betrieben werden. Eine Premium Lizenz ermöglicht die lokale Bereitstellung der Software.
Anmerkung: Sowohl die Pro als auch die Premium Lizenz umfassen weitere Leistungen, welche in Einzelfällen ähnlich bedeutend sein können.
Um nur einige wenige zu nennen:
- Eingebettete Dashboards auf Webseiten oder anderer SaaS Anwendungen
- Nutzung der Power BI mobile app
- Inkrementelle Aktualisierung von Datenquellen
- Erhöhung der Anzahl automatischer Aktualisierungen pro Tag (Pro = 8)
- u.v.m.
Community & Features von anderen Entwicklern
Power BI Benutzer können sich einer sehr großen Community erfreuen, da diese Software sich laut Gartner unter den führenden BI Tools befindet und Microsoft einen großen Kundenstamm vorzuweisen hat. Dementsprechend gibt es nicht nur auf der Microsoft eigenen Webseite https://community.powerbi.com/ eine Vielzahl von Themen, welche erörtert werden, sondern behandeln auch die einschlägigen Foren Problemstellungen und bieten Infomaterial an. Dieser große Kundenstamm bietet eine attraktive Geschäftsgrundlage für Entwickler von Produkten, welche komplementär oder gar substitutiv zu einzelnen Funktionen von Power BI angeboten werden. Ein gutes Beispiel für einen ersetzenden Service ist das Tool PowerBI Robots, welches mit Power BI verbunden, automatisch generierte E-Mails mit Screenshots von Dashboards an beliebig viele Personen sendet. Da dafür keine Power BI Pro Lizenz benötigt wird, hebelt dieser Service die wichtige Veröffentlichungsfunktion und damit einen der Hauptgründe für die Beschaffung der Pro Lizenz teilweise aus. Weiterhin werden Features ergänzt, welche noch nicht durch Microsoft selbst angeboten werden, wie z.B. die Erweiterung um ein Process Mining Tool namens PAFnow. Dieses und viele weitere Angebote können auf der Marketplace-Plattform heruntergeladen werden, sofern man eine Pro Lizenz besitzt.
Daten laden: Allgemeines
Ein sehr großes Spektrum an Datenquellen wird von Power BI unterstützt und fast jeder Nutzer sollte auf seinen Datenbestand zugreifen können. Unterstützte Datenquellen sind natürlich diverse Textdateien, SaaS verschiedenster Anbieter und Datenbanken jeglicher Art, aber auch Python, R Skripte sowie Blank Queries können eingebunden werden. Ebenfalls besteht die Möglichkeit mit einer ODBC-Schnittstelle eine Verbindung zu diversen, nicht aufgelisteten Datenquellen herstellen zu können. Ein wesentlicher Unterschied zwischen den einzelnen Datenquellen besteht in der Limitierung, eine direkte Verbindung aufsetzen zu können, eine sogenannte DirectQuery. In der Dokumentation zu Datenquellen findet man eine Auflistung mit entsprechender Info zur DirectQuery. Die Alternative dazu ist ein Import der Daten in Kombination mit regelmäßig durchgeführten Aktualisierungen. Mit Dual steht dem Anwender ein Hybrid aus beiden Methoden zur Verfügung, welcher in besonderen Anwendungsfällen sinnvoll sein kann. Demnach können einzelne Tabellen als Dual definiert und die im Folgenden beschriebenen Vorteile beider Methoden genutzt werden.
Import vs DirectQuery
Welche Verbindung man wählen sollte, hängt von vielen Faktoren ab. Wie bereits erwähnt, besteht eine Limitierung von 8 Aktualisierungen pro Tag und je Dataset bei importierten Datenquellen, sofern man nur eine Pro Lizenz besitzt. Mit der Nutzung einer DirectQuery besteht diese Limitierung nicht. Ebenfalls existiert keine Beschränkung in Bezug auf die Upload-Größe von 1GB je Dataset. Eine stetige Aktualität der Reports ist unter der Einstellung DirectQuery selbst redend.
Wann bringt also der Import Vorteile?
Dieser besteht im Grunde in den folgenden technischen Limitierungen von DirectQuery:
- Es können nicht mehr als 1 Mio. Zeilen zurückgegeben werden (Aggregationen wiederum können über mehr Zeilen laufen).
- Es können nur eingeschränkt Measures (Sprache DAX) geschrieben werden.
- Es treten Fehler im Abfrageeditor bei übermäßiger Komplexität von Abfragen auf.
- Zeitintelligenzfunktionen sind nicht verfügbar.
Daten laden: AdventureWorks2017Dataset
Wie zu erwarten, verlief der Import der Daten reibungslos, da sowohl die Datenquelle als auch das Dataset Produkte von Microsoft sind. Ein Import war notwendig, um Measures unter Nutzung von DAX anzuwenden. Power BI ermöglichte es, die Daten schnell in das Tool zu laden.
Beziehungen zwischen Datentabellen werden durch die Software entweder aufgrund von automatischer Erkennung gleicher Attribute über mehrere Tabellen hinweg oder durch das Laden von Metadaten erkannt. Aufgrund des recht komplexen und weit verzweigten Datasets schien dieses Feature im ersten Moment von Vorteil zu sein, erst in späteren Visualisierungsschritten stellte sich heraus, dass einige Verbindungen nicht aus den Metadaten geladen wurden, da eine falsch gesetzte Beziehung durch eine automatische Erkennung gesetzt wurde und so die durch die Metadaten determinierte Beziehung nicht übernommen werden konnte. Lange Rede kurzer Sinn: Diese Automatisierung ist arbeitserleichternd und nützlich, insbesondere für Einsteiger, aber das manuelle Setzen von Beziehungen kann wenig auffällige Fehler vermeiden und fördert zugleich das eigene Verständnis für die Datengrundlage. Microsoft bietet seinen Nutzer an, diese Features zu deaktivieren. Das manuelle Setzen der Beziehungen ist über das Userinterface (UI) im Register „Beziehungen“ einfach umzusetzen. Besonders positiv ist die Verwirklichung dieses Registers, da der Nutzer ein einfach zu bedienendes Tool zur Strukturierung der Daten erhält. Ein Entity-Relationship-Modell (ERM) zeigt das Resultat der Verknüpfung und zugleich das Datenmodel gemäß dem Konzept eines Sternenschemas.
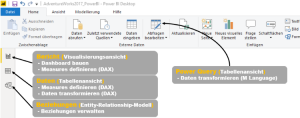
Daten transformieren
Eines der wesentlichen Instrumente zur Transformierung von Daten ist Power Query. Diese Software ist ebenfalls ein etablierter Bestandteil von Excel und verfügt über ein gelungenes UI, welches die Sprache M generiert. Ca. 95% der gewünschten Daten Transformationen können über das UI durchgeführt werden und so ist es in den meisten Fällen nicht notwendig, M schreiben zu müssen. Durch das UI ermöglicht Power Query, wesentliche Aufgaben wie das Bereinigen, Pivotieren und Zusammenführen von Daten umzusetzen. Aber es ist von Vorteil, wenn man sich zumindest mit der Syntax auskennt und die Sprache in groben Zügen versteht. Die Sprache M wie auch das UI, welches unter anderem die einzelnen Bearbeitungs-/Berechnungsschritte aufzeigt, ist Workflow-orientiert. Das UI ist gut strukturiert, und Nutzer finden schnellen Zugang zur Funktionsweise. Ein sehr gut umgesetztes Beispiel ist die Funktion „Spalten aus Beispielen“. In nur wenigen Schritten konnten der Längen- und Breitengrad aus einer zusammengefassten Spalte getrennt werden. Den erzeugten M-Code und den beschriebenen Workflow seht ihr in der folgenden Grafik.
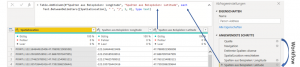
Das Feature zur Zusammenführung von Tabellen ist jedoch problematisch, da das UI von Power Query dem Nutzer keine vorprogrammierten Visualisierungen o.ä. an die Hand gibt, um die Resultate überprüfen zu können. Wie bei dem Beispiel Dataset von Microsoft, welches mit über 70 Tabellen eine relativ komplexe Struktur aufweist, können bei unzureichender Kenntnis über die Struktur der Datenbasis Fehler entstehen. Eine mögliche Folge können die ungewollte Vervielfachung von Zeilen (Kardinalität ist „viele zu viele“) oder gar das Fehlen von Informationen sein (nur eine Teilmenge ist in die Verknüpfung eingeschlossen). Zur Überprüfung der JOIN Ergebnisse können die drei genannten Register (siehe obige Grafik) dienen, aber ein Nutzer muss sich selbst ein eigenes Vorgehen zur Überwachung der korrekten Zusammenführung überlegen.
Nachdem die Bearbeitung der Daten in Power Query abgeschlossen ist und diese in Power BI geladen werden, besteht weiterhin die Möglichkeit, die Daten unter Nutzung von DAX zu transformieren. Insbesondere Measures bedienen sich ausschließlich dieser Sprache und ein gutes Auto-Fill-Feature mit zusätzlicher Funktionsbeschreibung erleichtert das Schreiben in DAX. Dynamische Aggregationen und etliche weitere Kalkulationen sind denkbar. Nachfolgend findet ihr einige wenige Beispiele, welche auch im AdventureWorks Dashboard Anwendung finden:
Measures können komplexe Formen annehmen und Power BI bietet eine sehr gute Möglichkeit gebräuchliche Berechnungen über sogenannte Quickmeasures (QM) vorzunehmen. Ähnlich wie für die Sprache M gibt es ein UI zur Erstellung dieser, ohne eine Zeile Code schreiben zu müssen. Die Auswahl an QM ist groß und die Anwendungsfälle für die einzelnen QM sind vielfältig. Als Beispiel könnt ihr euch das Measure „Kunden nach Year/KPI/Category“ im bereitgestellten AdventureWorks Dashboard anschauen, welches leicht abgewandelt auf Grundlage des QM „Verkettete Werteliste“ erstellt wurde. Dieses Measure wurde als dynamischer Titel in das Balkendiagramm eingebunden und wie das funktioniert seht ihr hier.
Daten visualisieren
Der letzte Schritt, die Visualisierung der Daten, ist nicht nur der wichtigste, sondern auch der sich am meisten unterscheidende Schritt im Vergleich der einzelnen BI-Tools. Ein wesentlicher Faktor dabei ist die Arbeitsabfolge in Bezug auf den Bau von Visualisierungen. Power BI ermöglicht dem Nutzer, einzelne Grafiken in einem UI zu gestalten und in dem selbigen nach Belieben anzuordnen. Bei Tableau und Looker zum Beispiel werden die einzelnen Grafiken in separaten UIs gestaltet und in einem weiteren UI als Dashboard zusammengesetzt. Eine Anordnung der Visualisierungen ist in Power BI somit sehr flexibel und ein Dashboard kann in wenigen Minuten erstellt werden. Verlieren kann man sich in den Details, fast jede visuelle Vorstellung kann erfüllt werden und in der Regel sind diese nur durch die eigene Zeit und das Know-How limitiert. Ebenfalls kann das Repertoire an Visualisierungen um sogenannte Custom Visualizations erweitert werden. Sofern man eine Pro Lizenz besitzt, ist das Herunterladen dieser Erweiterungen unter AppSource möglich.
Eine weitere Möglichkeit zur Anreicherung von Grafiken um Detailinformationen, besteht über das Feature Quickinfo. Sowohl eine schnell umsetzbare und somit wenig detaillierte Einbindung von Details ist möglich, aber auch eine aufwendigere Alternative ermöglicht die Umsetzung optisch ansprechender und sehr detaillierter Quickinfos.
Das Setzen von Filtern kann etliche Resultate und Erkenntnisse mit sich bringen. Dem Nutzer können beliebige Ansichten bzw. Filtereinstellungen in sogenannten Bookmarks gespeichert werden, sodass ein einziger Klick genügt. In dem AdventureWorks Dashboard wurde ein nützliches Bookmark verwendet, welches dem Zurücksetzen aller Filter dient.
Erstellt man Visualisierungen im immer gleichen Format, dann lohnt es sich ein eigenes Design in JSON-Format zu erstellen. Wenn man mit diesem Format nicht vertraut ist, kann man eine Designvorlage über das Tool Report Theme Generator V3 sehr einfach selbst erstellen.
Existiert ein Datenmodell und werden Daten aus verschiedenen Tabellen im selben Dashboard zusammengestellt (siehe auch Beispiel Dashboard AdventureWorks), dann werden entsprechende JOIN-Operationen im Hintergrund beim Zusammenstellen der Visualisierung erstellt. Ob das Datenmodell richtig aufgebaut wurde, ist oft erst in diesem Schritt erkennbar und wie bereits erwähnt, muss sich ein jeder Anwender ein eigenes Vorgehen überlegen, um mit Hilfe dieses Features die vorausgegangenen Schritte zu kontrollieren.
Warum braucht Power BI eine Python Integration?
Interessant ist dieses Feature in Bezug auf Machine Learning Algorithmen, welche direkt in Power BI integriert werden können. Python ist aber auch für einige Nutzer eine gern genutzte Alternative zu DAX und M, sofern man sich mit diesen Sprachen nicht auseinandersetzen möchte. Zwei weitere wesentliche Gründe für die Nutzung von Python sind Daten zu transformieren und zu visualisieren, unter Nutzung der allseits bekannten Plots. Zudem können weitere Quellen eingebunden werden. Ein Vorteil von Python ist dessen Repertoire an vielen nützlichen Bibliotheken wie pandas, matplotlib u.v.m.. Jedoch ist zu bedenken, dass die Python-Skripte zur Datenbereinigung und zur Abfrage der Datenquelle erst durch den Data Refresh in Power BI ausgeführt werden. In DAX geschriebene Measures bieten den Vorteil, dass diese mehrmals verwendet werden können. Ein Python-Skript hingegen muss kopiert und demnach auch mehrfach instandgehalten werden.
Es ist ratsam, Python in Power BI nur zu nutzen, wenn man an die Grenzen von DAX und M kommt.
Fazit
Das Lizenzmodel ist stark auf die Nutzung in der Cloud ausgerichtet und zudem ist die Funktionalität der Software, bei einer lokalen Verwendung (Power Bi Report Server) verglichen mit der cloud-basierten Variante, eingeschränkt. Das Lizenzmodell ist für den Power BI Neuling, welcher geringe Kapazitäten beansprucht einfach strukturiert und sehr transparent. Bereits kleine Firmen können so einen leichten Einstieg in Power BI finden, da auch kein Mindestumsatz gefordert ist.
Gut aufbereitete Daten können ohne großen Aufwand geladen werden und bis zum Aufbau erster Visualisierungen bedarf es nicht vieler Schritte, jedoch sind erste Resultate sehr kritisch zu hinterfragen. Die Kontrolle automatisch generierter Beziehungen und das Schreiben von zusätzlichen DAX Measures zur Verwendung in den Visualisierungen sind in den meisten Fällen notwendig, um eine korrekte Darstellung der Zahlen zu gewährleisten.
Die Transformation der Daten kann zum großen Teil über unterschiedliche UIs umgesetzt werden, jedoch ist das Schreiben von Code ab einem gewissen Punkt unumgänglich und wird auch nie komplett vermeidbar sein. Power BI bietet aber bereits ein gut durchdachtes Konzept.
Im Großen und Ganzen ist Power BI ein ausgereiftes und sehr gut handhabbares Produkt mit etlichen Features, ob von Microsoft selbst oder durch Drittanbieter angeboten. Eine große Community bietet ebenfalls Hilfestellung bei fast jedem Problem, wenn dieses nicht bereits erörtert wurde. Hervorzuheben ist der Kern des Produkts: die Visualisierungen. Einfach zu erstellende Visualisierungen jeglicher Art in einem ansprechenden Design grenzen dieses Produkt von anderen ab.
Fortsetzung: Tableau wurde als zweites Tool dieser Artikelserie näher beleuchtet.